Projects 研究プロジェクト
Projects
AIを活用した物質の量子的性質の解読 (Quantum ID -物質の「量子指紋」をAIで読み取り利用する-) Analysis of materials’ quantum properties using AI (Quantum ID: Exploiting “Quantum Fingerprintings” of materials with AI)
構成メンバー
People
- 研究リーダー Project Leader
-
東京大学 大学院工学系研究科 Graduate School of Engineering, The University of Tokyo
- 研究担当者 Researcher
-
-
沙川 貴大 教授 Takahiro Sagawa Professor
-
横井 直人 特任研究員 Naoto Yokoi Project Researcher
-
星 幸治郎 特任研究員 Koujiro Hoshi Project Researcher
-
Alexey Kaverzin 特任研究員 Alexey Kaverzin Project Researcher
-
- 研究協力者 Research Collaborator
- 橋本 幸士 Koji Hashimoto 皆川 麻利江 Marie Minagawa 吉岡 信行 Nobuyuki Yoshioka 日置 友智 Tomosato Hioki
Events
Events
- 第61回研究セミナー 「生成AIで読み解く量子カオス」 The 61st Research Seminar: "Understanding quantum chaos using generative AI"
- 第52回研究セミナー 「自動微分を用いた欲しい性質を持つ物理系の逆設計」 The 52nd Research Seminar: Inverse design of physical systems with desired properties using automatic differentiation
- 特別セミナー「Neural Polytopes」 Special Research Seminar: Neural Polytopes
- 第43回研究セミナー「AI でつなぐ古典世界と量子世界」 The 43rd Research Seminar: Connecting classical and quantum world with AI
News
News
- 【プレスリリース】磁石に隠されていた振動の情報を取り出すことに成功 ――磁気情報デバイス開発に道―― [Press Release] Extracting the hidden coherence within magnets - New possibilities of the development of magnetic information devices.
- 齊藤英治先生の研究グループからコラムがアップされました Professor Eiji Saitoh, leader of the Mid- and Long-term research project has posted a column regarding his research at the Institute for AI and Beyond
- [受賞] 中長期研究グループの齊藤英治教授が仁科記念賞を受賞 [Award] Professor Eiji Saitoh of the Mid- and Long-term Research Group awarded the Nishina Memorial Prize for 2022
- [プレスリリース] AIを利用し「量子指紋」を解読することに成功 Press Release: Researchers succeed in deciphering "quantum fingerprints" using AI (Artificial Intelligence).
ビデオ
Movie
課題
Challenges
量子物理現象の持つ複雑すぎる情報 Complex nature of information obtained from quantum systems
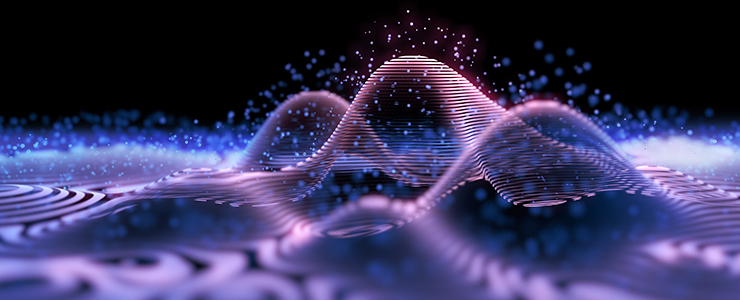
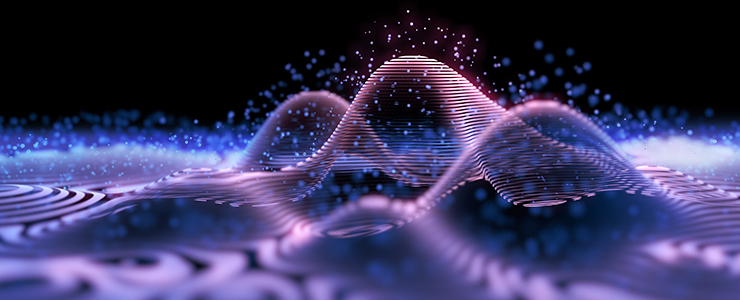
私たちの認識で捉えられるような古典物理学が支配するマクロスケールな世界と、ミクロなスケールで観察される量子力学が支配する世界は大きく異なっています。ミクロなスケールで起きる量子物性現象を対象にした計測技術は大きく進歩をしていますが、量子そのものや多体系と呼ばれる複数の自由度が影響を及ぼし合うような系で観察される現象はその信号の複雑さ故に、現状の技術や理論では正面から扱うことができません。この限界は、そもそも私たち人間が複雑過ぎる多次元の情報を概念的に理解することができないことと深く関係しています。しかし、例え人間には理解できなくとも、これらのデータはなんらかの物理情報を運んでいるはずです。そこで、急速に発展するAIを活用し、これまで理解不能であった量子物性を解読することで、人間にとって利用できるものに還元します。
The macroscopic world governed by classical physics, as we perceive it, differs vastly from the microscopic world governed by quantum physics. Despite the great strides that have been made in measurement technology for quantum properties, many phenomena observed in the quantum many-body systems, where multiple degrees of freedom influence each other, cannot be addressed directly with current methods due to the complexity of the signals. This limitation is closely related to the fact that we humans are incapable of conceptually comprehending information that is too complex and multi-dimensional. However, even if we cannot understand it, these data carries information of the quantum systems. Thus, the use of rapidly developing AI will facilitate the deciphering of previously incomprehensible quantum properties, and thereby reduce them to something that can be used by humans.
研究の内容
Details of Project
量子物理とAIの融合で新しい研究分野を開拓 Exploring new research fields by combining quantum physics and AI
量子系が示す複雑な信号を読み取り利用するためのAI物理科学の開発が本研究の目標です。従来の科学技術のほとんどの領域では、量子現象に現れる揺らぎをマクロに平均化して扱っており、ミクロの膨大な自由度はほとんど利用されていませんでした。ところがAIの登場により、ミクロ自由度の一部を積極的に利用し、人類が利用可能な物理情報を各段に拡張する方法が見出されました。これにより、量子性や非線形性といった量子の特性を利用した次世代のプロセッシングの実現、つまり情報の記録・演算などの機能をミクロな自由度を使って実装する全く新しいコンピューティングを開発できる可能性が高まっています。
This research aims to develop AI-physical science for reading and using the complex signals produced by quantum systems. Most classical science and technology deals with macroscopic averaging of fluctuations of signals obtained from quantum systems, rarely leveraging the vast microscopic degrees of freedom. However, the emergence of AI has enabled us to actively utilize some of the unused microscopic degrees of freedom and to transcribe it into information human beings can understand. This in turn has increased the possibility of establishing the next-generation processing utilizing various material properties such as quantum nature and nonlinearity, in other words, developing a completely new type of computing that implements functions such as recording and computing information using microscopic degrees of freedom.
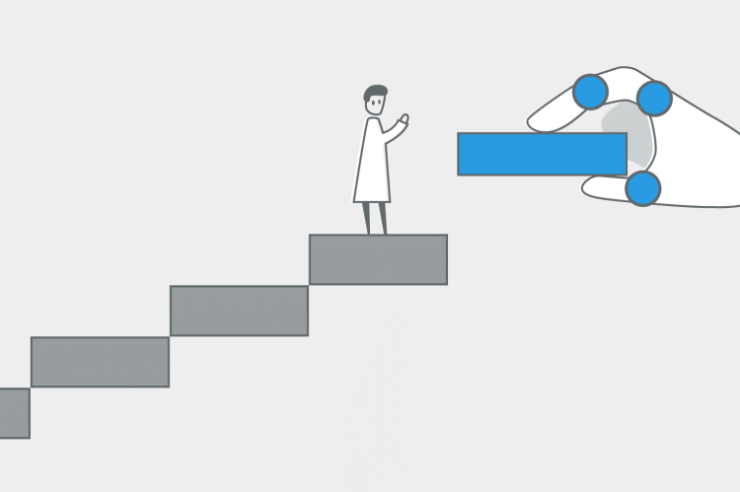
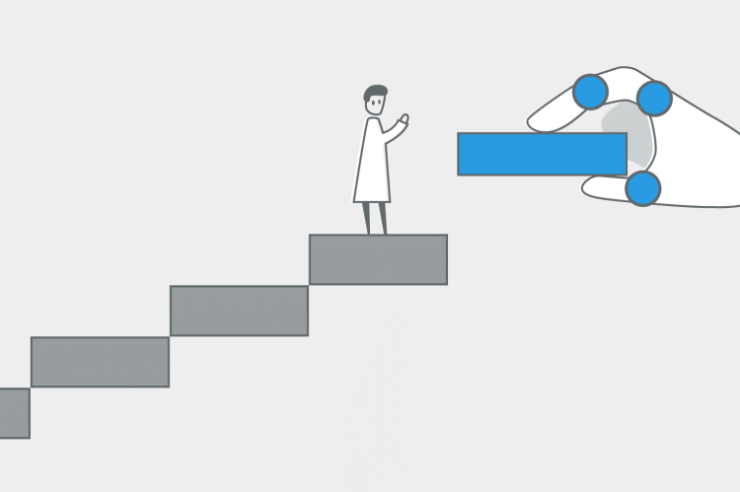
【1】AIにより量子の世界を解読する [1] Decoding the quantum world with AI
本研究では、まず“量子ビット”や“量子指紋”と呼ばれる量子の持つ複雑な出力信号を解読し、利用することを目指します。AI研究者と量子物性計測研究者が連携し、測定装置の思い切った融合を行えば、量子コンピューティング技術や量子物理タグ等への応用にもつながることが大いに期待できます。つまり、現実世界を人間が理解できる自由度を超えた複雑な量子の世界に写し取ることが可能になり、ひいては量子人工知能によって人類の認識や考え方および科学技術そのものが拡張されることにつながります。
In this research, we first aim to decipher and utilize complex quantum output signals called “quantum fingerprints” and “quantum bits (qubits).” AI scientists and researchers in the field of quantum physical property measurement collaborate and boldly integrate their measurement devices, contributing to applications such as quantum computing technology and quantum physical tags. In other words, it would allow for mapping the real world into a complex quantum world beyond the degrees of freedom that humans can comprehend, which in turn would help expand human perception, thinking, and science and technology itself through quantum AI.
【2】量子・AI両者の強みを活かしてイノベーションの連鎖を生む [2] Harnessing the strengths of both quantum and AI to trigger a chain reaction of innovation
これまでの研究から、量子物性や量子科学技術分野で世界をリードする学術知見とノウハウが蓄積されています。培ってきた量子物理学実験における高度な計測技術と、機械学習における情報処理の2点がそれぞれの強みで補い合うことで、両分野の発展を加速させます。既にこれまでの研究成果で、ミクロ自由度がもたらす物理現象の複雑性を深層学習の手法を用いることで、情報資源として利用できるように変換できることが分かってきています。研究をさらに推進し、常識にとらわれない挑戦とイノベーションの連鎖を引き起こします。
Through our research to date, we have accumulated world-leading academic knowledge and expertise in the fields of quantum properties and quantum science and technology. We will accelerate the further progress of both areas by supplementing the respective strengths of our two research fields: advanced measurement technology in quantum physics experiments and information processing in machine learning. Our existing studies have demonstrated that the complexity of physical phenomena arising from microscopic degrees of freedom can be converted into usable information resources using the deep learning methods. We will further pursue our research to trigger a chain reaction of unconventional challenges and innovations.
価値・期待
Values / Hopes
量子を利用した新しい技術開発で科学を変える Changing science through the development of new quantum-based technologies
量子の世界と私たちの現実世界の間をつなげるルートを、AIを用いて発見することで、科学全体が変わり、量子を利用した新しい技術開発が可能になると期待されます。例えば、物質の同一性を究極的に決める手法が開発できれば、製品などあらゆるものを識別する“量子物理タグ”として実社会への応用も可能です。そして、量子物理と人工知能の融合に対する期待として、量子の複雑性・非線型性を積極的に人工知能に取り入れた量子人工知能分野が開拓されたり、一方でAIを活用することで量子が持つゆらぎを高度に制御することで新たな量子コンピューティング技術が開発できたりと、両分野にイノベーションが引き起こされると予想されます。
Discovering a route between the quantum world and our real world through AI would transform science, allowing for the development of new quantum-based technologies. For example, if we can develop a method to ultimately determine the identity of substance, it could be applied to the real world as a “quantum physics tag” to identify products and many other things. In the future, the combination of quantum physics and AI will be likely to make innovation happen in multiple areas: the exploration of the field of quantum AI, in which the complexity and non-linearity of quantum are actively incorporated into AI; and the development of new quantum computing technology, in which the fluctuations of quantum are controlled to a high degree through the use of AI.
成果
Research outcome
本プロジェクトにおける研究目標は、量子力学で支配されるミクロな物理系に現れる複雑な量子物性や量子系からの信号に潜む情報のAIを用いた抽出です。
第一に、微小な金属中の電気伝導に現れる電気抵抗の複雑な揺らぎのAIを用いた解析です。たとえば、ナノメートルスケールの非常に微小な金属中では、電気伝導を担う電子は量子力学の法則に従い、粒子であるとともに波動としての性質を併せ持ち、波の干渉と類似した量子干渉現象を示します。微小金属中に原子レベルの不純物や格子欠陥などが存在すると、それらによって散乱された波同士や金属端からの反射波との干渉などにより、金属に印加する磁場の大きさの変化に応じて、非常に複雑な揺らぎのパターンを示します。この複雑なパターンは、本質的に量子力学由来のもので、古典世界のランダムな熱揺らぎなどとは異なり、微小金属中の不純物の位置などのミクロな情報を保持しており、「量子指紋」と呼ばれています。しかしながら、従来は、その複雑さゆえにそこに潜むミクロな情報を読み取ることは困難でした。本研究では、生成AIを用いることにより、電気抵抗に現れる量子指紋から金属中の波動関数の量子干渉や電子構造の情報を抽出することに成功しました。具体的には、様々な不純物の配置を持った金属に対して電子のシュレーディンガー方程式を数値計算を用いて解くことにより、金属中の波動関数の干渉パターンとそれから得られる電気抵抗の磁場依存性を求め、まず波動関数の干渉パターンの画像データを変分自己符号化器(VAE)等を用いて学習し、波動関数の特徴量を潜在空間に抽出しました。次に、電気抵抗の揺らぎである量子指紋のデータから、VAEで抽出した干渉パターンの特徴量を推定するネットワークを学習し、それぞれの金属の量子指紋から不純物の位置や波動関数などのミクロな量子情報を再生成することに成功しました。
また、量子コンピュータの出力に現れる揺らぎ(エラー)に関するAIを用いた研究も行いました。量子コンピュータの基本単位である量子ビットに対する操作は量子力学で支配されますが、様々な外部からの擾乱により、正確な量子操作を実現するのが非常に困難であることが知られています。量子計算に必要な量子操作の数の増大に応じてエラーの頻度も上昇し、出力結果に対する揺らぎが大きくなり、意味のある計算が出来なくなります。本プロジェクトでは、量子計算に使用される量子回路(量子操作の列)に関して、理想的に期待される入力と出力の間の関係に対するAIを用いた強化学習を行うことにより、従来から知られていた量子回路と(ほぼ)同じ出力を実現する、ノイズに強い短い量子操作で構成された新しい量子回路を発見しました。発見された量子回路は従来の量子回路よりも量子操作の数が少ないため、最終的な出力に対するエラーも小さくなると考えられ、この新しい量子回路を超伝導量子ビットを用いた実際の量子コンピュータにおいて実装することにより、従来よりもエラーの小さい量子計算の出力結果を得ることに成功しました。
上記のような量子性を示すと既に知られている物理系に対するAIを用いた研究に加えて、本プロジェクトではAIの研究対象となる新たな量子物理系の探索も行っています。その一つが磁性体中の素励起であるマグノンです。マグノンは磁性を担う電子スピンのゆらぎであり、スピンを用いた次世代省電力素子を研究するスピントロニクスと呼ばれる研究領域において重要な役割を果たしてきました。マグノンを量子情報担体として用いるには、大量のデータ取得によるマグノン揺らぎの解析が必要となります。本研究では、状態トモグラフィと呼ばれる手法をマグノンに対して初めて実現し、磁場やマイクロ波などによって生成・制御されたマグノンの状態が持つ量子性の有無を判別することが可能となりました。
※詳細については、以下のURL(PDF・英文のみ)をご参照ください
https://beyondai.jp/contents/wp-content/uploads/2024/02/Research-report_Eiji-Saitoh_202309.pdf
The research objective of this project is to extract information hidden in complex quantum properties and quantum fluctuations, which appear in microscopic physical systems governed by quantum mechanics, using artificial intelligence (AI).
Firstly, we aim at the AI-assisted analysis of complex fluctuations in electrical resistance that manifest in microscopic electrical conduction in metals. In the minuscule metals with the nanometer size, the electrons responsible for electrical conduction obey quantum mechanical law, demonstrating properties of both particles and waves, leading to quantum interference phenomena similar to the interference of water waves. The atomic-level impurities and lattice defects in the micro-metal lead to the interference between the wave functions, which are scattered from the impurities (or defects) and reflected at the edges of the metal. As a result, when the magnitude of the magnetic field applied to the metal changes, the electrical resistance exhibits a highly complex pattern of fluctuations in response. This complex pattern is essentially originated from quantum mechanics, and unlike the random thermal fluctuations of the classical world, it retains microscopic information such as the positions of impurities in micro-metals, thus being termed as a 'quantum fingerprint'. However, due to its complexity, the standard procedure has been to analyze these fluctuation patterns by averaging, making it challenging to decode the hidden microscopic information. In this research, we successfully managed to extract information about quantum interference of wave functions in micro-metals from the quantum fingerprints using AI. More specifically, we dealt with micro-metals incorporating various impurity arrangements by numerically solving the Schrödinger equation for electrons, allowing us to determine interference patterns of the wave functions and derive the magnetic field-dependent electrical resistance. We start with the machine learning on image data of these interference patterns based on a Variational Autoencoder (VAE), enabling us to extract their characteristic features in a latent space. Subsequently, we trained a network to estimate the characteristic features extracted by the VAE from the quantum fingerprints in electrical resistance. This approach enabled us to successfully reconstruct microscopic quantum information such as impurity positions and interference patterns from the quantum fingerprint in the micro-metal.
Next, we utilize AI in research on fluctuations that appear in the output of quantum computations performed by quantum computers and lead to errors in computations. Quantum operations performed on quantum bits (qubits), the basic units of a quantum computer, are governed by quantum mechanics, and it is known to be extremely challenging to achieve precise quantum operations due to various external disturbances. The frequency of errors increases with the number of quantum operations required for quantum computation, leading to greater fluctuations in the output results which make meaningful computations impossible. In this project, we performed reinforcement learning in AI for the ideal relationship between inputs and outputs for the quantum circuits (sequences of quantum operations) used in quantum computations. This allowed us to discover new quantum circuits that achieve (almost) the same outputs as well-known quantum circuits but are composed of fewer quantum operations. The discovered quantum circuit, with fewer quantum operations compared to conventional ones, can exhibit smaller errors in the final outputs. Implementing this new quantum circuit using superconducting qubits in an actual quantum computer has successfully led to output results with fewer errors compared to conventional circuits in quantum computations.
We are conducting research using AI not only for known physical systems that exhibit quantum properties, but also exploring new quantum physical systems for the AI-assisted research in this project. One of these is the system of magnons, which are elementary excitations in magnetic materials. Magnons are fluctuations in the quantum spins of electrons responsible for magnetism and have played a crucial role in the field of spintronics, where next-generation, low-power-consumption devices utilizing spins are actively explored. The utilization of magnons as quantum information carriers necessitates the analysis of magnon fluctuations through extensive data acquisition. In this study, we have successfully implemented a technique called state tomography for the first time concerning magnons, allowing the determination of the presence or absence of quantum properties in the states of magnons generated and controlled by magnetic fields and microwaves.
※For more detail, please refer to the URL below.
https://beyondai.jp/contents/wp-content/uploads/2024/02/Research-report_Eiji-Saitoh_202309.pdf