Projects 研究プロジェクト
Projects
量子ゆらぎから天の川銀河の形成史の解明を通じたAIの進展 New developments in AI through study of evolution of the Milky Way from initial quantum fluctuations to its assembly
構成メンバー
People
- 研究リーダー Project Leader
-
東京大学 国際高等研究所カブリ数物連携宇宙研究機構 Kavli Institute for the Physics and Mathematics of the Universe, (Kavli IPMU), The University of Tokyo
Events
Events
- 第56回研究セミナー 「すばるデータによる宇宙論と最近のMLの宇宙論への応用の話題」 The 56th Research Seminar: Cosmology results with Subaru HSC data and recent topics on ML applications to cosmology
- 第7回 Beyond AI 研究推進機構サイエンスカフェ 動画を公開 The 7th Institute for AI and Beyond Science Café Video is now available.
- 第31回研究セミナー「Our universe in simulation」 The 31st Research Seminar: Our universe in simulation
- 第15回研究セミナー「宇宙のビックデータと機械学習」 15th Research Seminar: Cosmic big data and ML/AI
News
News
- 【プレスリリース】ダークマターを見る!– HSC国際チームが宇宙の標準理論を検証 [Press Release] How to see the invisible: Using dark matter distribution to test our cosmological model
- [プレスリリース] AIとスーパーコンピュータで広大な銀河地図を解読 – 宇宙の成り立ちを決める物理量を精密に測定 [Press Release] Extensive training on virtual universes from supercomputer simulations produces AI-assisted analysis of three-dimensional galaxy distribution in our Universe
ビデオ
Movie
課題
Challenges
天文ビッグデータのAI解析からから天の川河銀河から宇宙の大規模構造の起源を探る Exploring the origins of the Milky Way and large-scale structures in the universe by AI-assisted analysis of astronomical big data
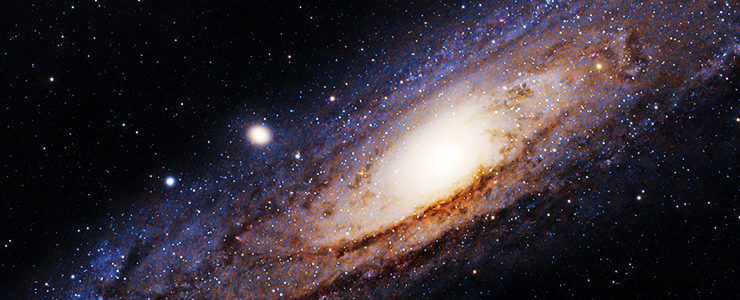
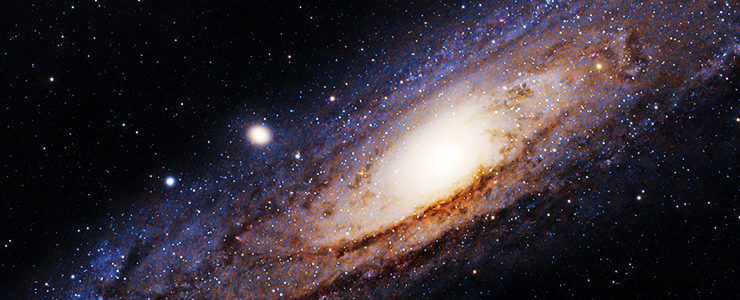
宇宙には、太陽、銀河、銀河団、また銀河分布の網の目構造(大規模構造)の豊かな階層構造があります。これら宇宙構造は、非常に面白い、また不思議なシナリオで形成されたと考えられています。まず、宇宙がバクテリアよりも小さかった宇宙極初期に、量子力学の不確定性関係が予言する“量子ゆらぎ”が宇宙の始まりの大爆発“インフレーション”とよばれる急激な膨張で引き延ばされ、宇宙構造の種を作りました。その後、正体不明のダークマターの重力で、その種ゆらぎが成長し、ダークマターが集合した領域に、星、銀河が作られ、銀河が合体・吸収を繰り返し、より大きな宇宙構造を作ってきました。さらに、“最近”の宇宙(約70億年前)では、これも謎のダークエネルギーによる加速膨張が始まり、構造形成の成長を妨げてきたというシナリオです。すばる望遠鏡をはじめとする天文ビックデータに機械学習・AIの手法を適用し、我々の住む天の川銀河から宇宙の大規模構造の起源を明らかにし、ダークマター、ダークエネルギーの謎に迫るのが本研究の目的です。
私たちが住む天の川銀河は、無数にある銀河のなかでも特別かつ唯一な存在であり、その起源を調べることは天文学の最も重要な研究テーマの一つです。天文学データの進展、計算機性能の向上、機械学習・AIなどの高度な統計的な技術の発展により、10億個にも及ぶ星のビッグデータを用いて天の川銀河の起源を探る研究が世界中で進展しています。また、これは宇宙の始まりの“量子ゆらぎ”や、宇宙を構成する物質の約86%を占めると言われる“ダークマター”の存在・性質といった、同じく重大な謎とも密接に関連しており、広く学術分野への貢献が期待できます。
The universe has a vast hierarchical structure spanning from suns, galaxies, clusters of galaxies, to web-like structures (large-scale structures) seen via galaxy distribution. These cosmic structures are believed to have formed in a most intriguing and mysterious scenario. At the very beginning of the universe, when the universe was no bigger than a bacterium, “quantum fluctuations” predicted by the uncertainty principle of quantum theory were stretched out by a rapid expansion called “inflation,” an accelerating cosmic explosion, to generate the seeds of cosmic structures. Then, the gravitational force of mysterious “dark matter” amplifies the primordial seeds fluctuations, driving cosmic structure formation. In this process small-scale structures such as stars or small galaxies first form in places where dark matter clusters and then larger-scale cosmic structures hierarchically form as a result of mergers and accretions of smaller structures. In the “recent” (about 7 billion years ago) universe, another accelerated expansion began due to another mysterious component, “dark energy”, which has hindered the growth of cosmic structures in the late universe. By applying machine learning and AI methods to astronomical big data such as those taken by the Subaru Telescope and other telescopes, our research aims to reveal the origins of cosmic structures of the universe ranging from the Milky Way we live in to large-scale structures, and to unveil the nature of dark matter and dark energy.
Our Milky Way Galaxy is a very special one because we live there, compared to other billions galaxies in the universe. How did the Milky Way form? – this question has always been one of astronomy's foremost research topics. Thanks to the advancement of astronomical data, enhancement of computer performance, and the development of advanced statistical techniques such as machine learning and AI, research on the origin of the Milky Way using big data of up to one billion stars has been facilitated throughout the world. Such research is also closely related to other important mysteries of the universe, including “quantum fluctuations” that were generated at the beginning of the universe and the existence and properties of “dark matter” accounting for about 86% of matter in the universe, and is expected to give an important contribution to interdisciplinary fields.
研究の内容
Details of Project
観測される膨大なデータとAIを用いて宇宙の謎に迫る Exploring the mysteries of the universe using vast amounts of observed data and AI
私たちの住む天の川銀河、銀河団、宇宙の大規模構造などの宇宙構造はどうやって出来たのか?ダークマター、ダークエネルギーはどのような影響を及ぼしてきたのか? これらは物理学・天文学における最も重要な問題です。現在考えられている標準的なシナリオは、宇宙初期の量子ゆらぎが種となり、その後ダークマターの重力の影響で進化・成長し、星を作り、それら星の集団が合体を繰り返し、天の川銀河をはじめとする宇宙の構造が形成されてきたというものです。観測によって得られた膨大な天文データをAIや機械学習の手法を用いることで、天の川銀河の成り立ち、宇宙構造の形成過程、またダークマター、ダークエネルギーの影響、さらには宇宙の初期条件の量子ゆらぎの性質について、新たな知見が得られると期待できます。
How did structures in the universe, such as our Milky Way, galaxy clusters, and large-scale structures, form? What impact have dark matter and dark energy had on the universe? They are among the most important questions in modern physics and astronomy. At present, the standard scenario is that the seed primordial fluctuations, originating from quantum fluctuations in the early universe, grow due to attractive gravitational force of dark matter, then form stars and clusters of stars, and eventually form the present-day structures including the Milky Way as a result of mergers and accretion of smaller structures. By applying AI and machine learning methods to the vast amount of astronomical data obtained from observations, we hope to gain new insights into the origin of the Milky Way, formation processes of cosmic structures, influences of dark matter and dark energy, and the nature and properties of quantum fluctuations in the early universe.
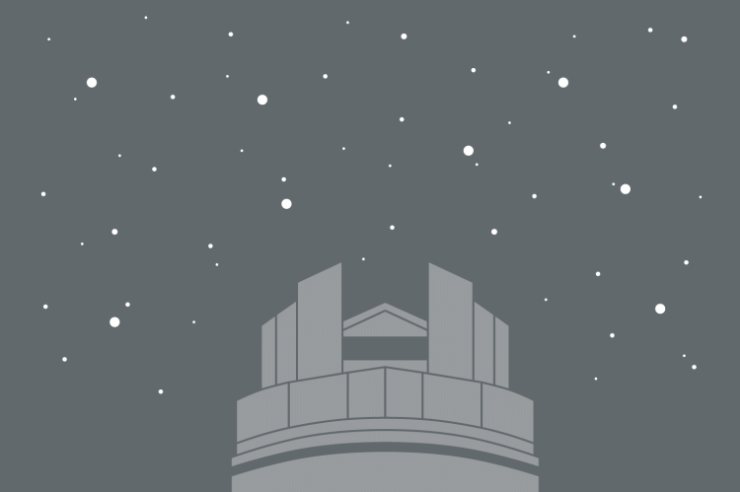
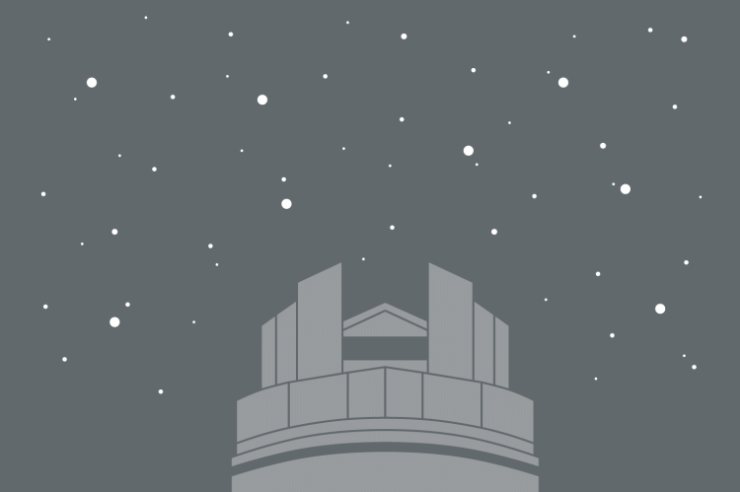
【1】天の川銀河は内部構造を観測できる唯一の天体 [1] The Milky Way is the only celestial body whose internal structure can be observed
天の川銀河は唯一観測によってその内部構造(星、ガスなどの分布、その物理状態)を詳しく調べることができる天体です。研究リーダーの村山が所属するカブリIPMUは、世界最大のカメラであるすばる望遠鏡Hyper Suprime-Cam (HSC)国際プロジェクトをリードしてきた主要研究機関です。すばる望遠鏡の集光力、シャープな画像のすばるHSCデータを活用するとともに、天の川銀河の3次元地図を作ることを目的に打ち上げられたGaia衛星から得られる星一つ一つの位置と速度の計6次元の位相空間情報を最大限利用します。
The Milky Way is only the galaxy whose internal structures (distribution of stars, gas, etc., and their physical states) can be studied in great detail by observations. The Kavli Institute for the Physics and Mathematics of the Universe (Kavli IPMU), to which leader Murayama belongs, is a major research institute that has been leading the international Hyper Suprime-Cam (HSC) project, the world's largest camera. For our research program, we use not only the Subaru Telescope's light-gathering power, the sharp images of the Subaru HSC data, but also make full use of the six-dimensional phase space information on the position and velocity of each star obtained from the Gaia satellite, launched for the purpose of creating a three-dimensional map of the Milky Way.
【2】天文ビックデータをAIで分析し、宇宙の構造形成の物理に迫る星々の運動を遡る [2] AI analysis of astronomical big data traces back the motions of the stars to understand the physics of the formation of cosmic structure
すばる望遠鏡のデータを始め、宇宙を調べる天文のビックデータは世界中の天文学者に共有され、宇宙の謎を解明するために使われています。このビックデータから余すことなく物理情報を引き出すことが喫緊の課題となっており、機械学習・AIの手法が強力になると期待されています。本研究では、この天文ビックデータを解析するためのAI手法を開発し、以下の研究を行います。宇宙の大規模構造の性質・進化を調べ、ダークマター、ダークエネルギーの情報を引き出し、その正体に迫ります。我々の天の川銀河の個々の星々の空間分布と運動を調べることで、天の川銀河のダークマターの情報(総量、分布)を調べます。また個々の星の空間分布と運動から、逆にその星々の運動を時間で遡ることにより、天の川銀河の起源に迫ります。さらに、ダークマターがその重力に支配的と考えられている矮小銀河(天の川銀河の衛星銀河)からのダークマター対消滅ガンマ線を探り、ダークマターの正体に迫ります。
Astronomers all over the world are sharing astronomical big data, including data from the Subaru Telescope, and are using it to uncover mysteries of the universe. Extracting the full range of physical information from this big data has become an urgent issue, and machine learning and AI methods are anticipated to contribute significantly to this effort. In this research, we will develop AI-assisted methods to analyze this astronomical big data, and carry out the following research. We aim to investigate the nature and evolution of the large-scale structures of the universe, and extract information on the nature of dark matter and dark energy. We will examine the information (total amount and distribution) of dark matter in the Milky Way by studying the spatial distribution and motions of individual stars in great detail. In addition, by tracing back motions of individual stars in time, we will explore the formation history and origin of the Milky Way. Furthermore, we will explore the nature of dark matter from a search of gamma-ray signals originating from annihilation of dark matter in dwarf galaxies (satellite galaxies of the Milky Way), where dark matter is thought to dominate the gravitational field.
価値・期待
Values / Hopes
天文物理学のアプローチとAIの融合で、人類共通の問いに答える Combining astrophysical approaches and AI to answer questions common to humanity
天の川銀河・宇宙構造の起源やダークマター、ダークエネルギーの正体、宇宙の起源・運命は、人類が長年挑んできた根本的な問題です。得られた結果は、一般講演などを通して積極的に社会に還元します。また、本研究では、量子ゆらぎ、重力の性質などの物理法則、世界最先端の観測データに基づき、「原因 (初期条件)」、「過程」、「結果」を論理的につなげ、系統的に問題を調べるアプローチを取ります。このような物理学の考え方とデータサイエンスおよび機械学習・AIという強力なツールを融合させることで、問題解決への新たな方法論の提案につなげるとともに、そうした技術を備えた高度な人材を育成し、社会に貢献することを目指します。
The origin of the Milky Way, cosmology, and the nature of dark matter and dark energy, as well as the origin and fate of the universe, are fundamental questions that humanity has been trying to answer for centuries. The results obtained will be actively returned to society through general lectures and public outreach. In this research, we adopt a systematic approach to investigate the problem by logically connecting “cause (initial conditions),” “process,” and “result” based on physical laws such as quantum fluctuations, the nature of gravity, and the world's cutting-edge observation data. By combining this approach with physics using powerful tools such as data science, machine learning, and AI, we aim to propose new methodologies for solving the problems, and to contribute to society by producing highly skilled human resources trained in such techniques.
成果
Research outcome
すばる望遠鏡をはじめとする銀河の分布を観測して得られる宇宙地図を用いた宇宙論の研究を進めることができました。宇宙地図から得られる宇宙論観測量と宇宙の標準理論であるΛCDM模型の理論予言を比較することにより、ダークマターの総量などの宇宙の物理量を測定することができます。スーパーコンピュータを用いた宇宙論的シミュレーションは、インフレーションの量子ゆらぎを起源とする物質分布のゆらぎを初期条件として、ダークマターの分布(物質分布の非一様性)の進化を正確に計算することが可能にします。ただし、スーパーコンピュータを使っても、一つの宇宙模型について高精度のシミュレーションを行うには数日を要します。
本研究では、スーパーコンピュータを用いて、100個の宇宙論モデルの各々について高精度の宇宙論シミュレーションを実行しました。そのシミュレーションデータをディープラーニングで学習し、シミュレーションを走らせていない宇宙モデルについても高精度シミュレーションと同程度の正確さをもつ構造形成の特性を予言できる機械「ダークエミュレータ」を作成しました。ダークエミュレータはスーパーコンピュータが数日で行うシミュレーションと同等の計算をたった0.1秒程度で行うことができます。つまり、我々は約100万倍の高速化に成功しました。このダークエミュレータで計算した理論予言と宇宙地図データから観測した宇宙論統計量を比較することで、宇宙論パラメータ、例えばダークマターの総量などを測定できました。これは宇宙地図データとスーパーコンピュータで計算した宇宙を比較するのと同等の手法になります。
最近では、すばる望遠鏡の主焦点カメラHype Suprime-Cam (HSC)のデータを用いた宇宙論成果を発表しました。すばるHSCのデータからアインシュタインが予言した弱重力レンズ効果を測定し、上記のダークエミュレータと比較することで、宇宙論パラメータを測定しました。
上述の我々の宇宙論パラメータの測定結果はどちらも独立な宇宙背景放射から得られた結果と若干の矛盾を示しており、業界で問題になっています。宇宙の新しい物理を示している可能性もあり、さらなる研究を進めているところです。宇宙ビッグデータを用いた宇宙論にAI/機械学習を用いた手法が今後益々用いられるのは間違いありません。
※詳細については、以下のURL(PDF・英文のみ)をご参照ください。
https://beyondai.jp/contents/wp-content/uploads/2024/06/Research-report_Murayama-Takada_202403.pdf
One of the most powerful cosmological methods is to use the map of the universe obtained from the distribution of galaxies that are observed by telescopes including the Subaru Telescope. By comparing cosmological observables obtained from the universe map with the predictions obtained from the standard model of the universe, we can measure the physical quantities of the universe such as the amount of dark matter. Cosmological simulations using supercomputers make it possible to accurately calculate the time evolution of the distribution of dark matter (the inhomogeneities of matter distribution) starting from the seed fluctuations in the early universe as the initial conditions. However, even with a supercomputer, it takes a few days to run a high-precision simulation for a single cosmological model.
In this project, we performed high-precision cosmological simulations for each of the 100 cosmological models using the supercomputer. By using a deep learning method to learn the simulation data, we were able to build "Dark Emulator," a machine that can predict the properties of structure formation with the same degree of accuracy as a high-precision simulation, even for an input cosmological model for which no simulation has been run. Dark Emulator can perform calculations in only about 0.1 second, which are equivalent to simulations that would take a few days to complete on a supercomputer. In other words, we were able to speed up the computation by a factor of 1 million. By comparing the theoretical predictions computed by Dark Emulator with the cosmological statistics observed from the universe map data, we were able to measure cosmological parameters such as the total amount of dark matter (Fig. 1). This method is equivalent to comparing the universe map with the universe computed by a supercomputer using different cosmological models.
Recently, we presented the cosmology results using the data obtained from the Hyper Suprime-Cam (HSC) on the Subaru Telescope in Hawaii. We measured the weak gravitational lensing effect, the prediction of the Einstein’s theory of gravity, from the Subaru HSC data and then compared the measurement with the predictions computed by Dark Emulator to measure the cosmological parameters.
Both of our cosmological parameter measurements mentioned above show some discrepancies with the independent results obtained from the cosmic microwave background data. This discrepancy is currently one of the hottest topics in the cosmology field. It may indicate new physics of the universe, and further research is underway. There is no doubt that AI/machine learning methods will be used more and more in cosmology, taking advantage of upcoming big data such as that from the Subaru Telescope.
※For more detail, please refer to the URL below.
https://beyondai.jp/contents/wp-content/uploads/2024/06/Research-report_Murayama-Takada_202403.pdf