Projects 研究プロジェクト
Projects
限られた教師情報からの高精度な予測モデルの自動構築に関する研究 Automatic Learning of High Accurate Prediction Models from Limited Supervised Data
構成メンバー
People
- 研究リーダー Project Leader
-
東京大学 先端科学技術研究センター Research Center for Advanced Science and Technology, The University of Tokyo
- 研究担当者 Researcher
-
-
杉山 将 教授 Masashi Sugiyama Professor
-
佐藤 一誠 教授 Issei Sato Professor
-
伊藤 信貴 特任講師 Nobutaka Ito Project Lecturer
-
長 隆之 准教授 Takayuki Osa Associate Professor
-
蔣 兆凱 特任助教 Chao-Kai Chiang Project Assistant Professor
-
Thomas Westfechtel 特任助教 Thomas Westfechtel Project Assistant Professor
-
黒瀬 優介 特任講師 Yusuke Kurose Project Lecturer
-
- 研究協力者 Research Collaborator
- 張 徳軒 Dexuan Zhang 張 一凡 Yivan Zhang 横井 創磨 Soma Yokoi 横矢 直人 Naoto Yokoya 石田 隆 Takashi Ishida Reza Motallebi Reza Motallebi 蔡 欣強 Xin-qiang Cai 房 彤彤 Tongtong Fang 張 宇傑 Yu-jie Zhang 陳 洪瑞軒 Hongruixuan Chen 王 維 Wei Wang 唐 玉亭 Yuting Tang
Events
Events
- 第60回研究セミナー 「Weakly Supervised Learning from a Unified Perspective」 The 60th Research Seminar: Weakly Supervised Learning from a Unified Perspective
- 特別セミナー「Toward Dense 3D Reconstruction in the Wild」 Special Research Seminar: Toward Dense 3D Reconstruction in the Wild by Dr. Jia Deng
- 第47回研究セミナー「ロボット学習における多様な解の発見」 The 47th Research Seminar: Discovering diverse solutions in robot learning
- 特別ゲストセミナー「Making the Invisible Visible: Towards High-Quality Physics-Guided THz Computational Imaging」 Special Guest Seminar "Making the Invisible Visible: Towards High-Quality Physics-Guided THz Computational Imaging"
News
News
- [受賞] 伊藤信貴特任講師と杉山将教授との論文が、ICASSP2023においてベストペーパーアワードを受賞 [Award] Dr. Nobutaka ITO (Project Lecturer) and Professor Masashi SUGIYAMA won the Best Paper Award at ICASSP 2023
- [受賞]原田達也教授が令和4年度 文部科学大臣表彰・科学技術賞(科学技術振興部門)を共同受賞されました。 [Awards]Professor Tatsuya HARADA jointly received the Awards for Science and Technology in Science and Technology Development Category
- [受賞] 杉山将教授が「令和4年度科学技術分野の文部科学大臣表彰」を受賞されました。 [AWARDS] Professor Masashi SUGIYAMA was selected for the 2022 Commendation for Science and Technology by the Minister of Education, Culture, Sports, Science and Technology.
ビデオ
Movie
課題
Challenges
教師データの整備という機械学習の導入障壁 Development of Training Data: The Barrier to Introduction of Machine Learning
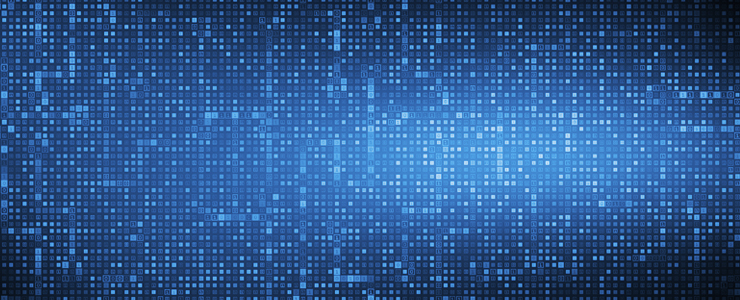
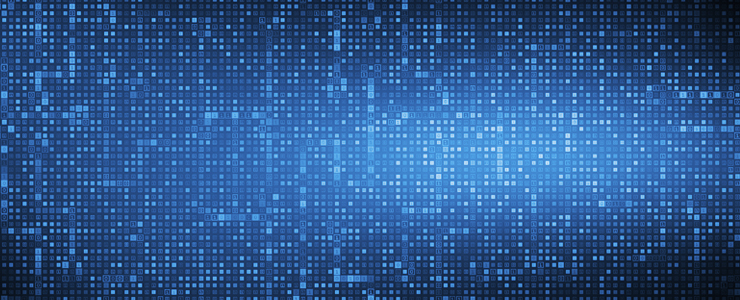
現在、機械学習による高度な予測機能を備えたシステムやサービスが急速に広がりつつあり、大きな注目を集めています。しかし機械学習、その中でもとりわけ深層学習の導入には教師データの整備という大きな障壁があることをご存知でしょうか?現在、深層学習の導入に成功しているのは主に「教師あり学習」と呼ばれる分野であり、この「教師あり学習」を利用して高い予測精度を得るには膨大な「教師データ」(=正解付きデータ)を準備する必要があります。多くの応用場面では新たに機械学習を導入しようとした際にこの「教師データ」を充分に用意することが出来ず、その恩恵にあずかれないという状況があります。また、仮にデータが入手可能であっても、教師データの整備には膨大なコストや専門的な知識が必要となり、導入の大きな障壁になっています。そこで、限られた情報でも機械学習を導入できるようにするための仕組みや、良質な教師データの整備にかかるコストの低減が、知的なシステムが世の中で汎用的に利用されるための最重要課題となっています。
※詳細については、以下のURL(PDF・英文のみ)をご参照ください。
Systems and services equipped with sophisticated prediction functions based on machine learning are rapidly expanding, attracting substantial interest. But did you know that there exists a huge barrier of training data development to the introduction of machine learning, particularly deep learning? Currently, deep learning is successfully introduced, primarily in the area of “supervised learning.” However, there is a need to prepare massive amounts of “training data” (data with correct answers) to achieve high prediction accuracy using the supervised learning. In numerous applications, sufficient training data cannot be prepared when attempts are made to introduce new machine learning processes, which may exclude developers and users from the benefits of training data. Even if the data required for building training data were available, the preparation process would require enormous cost and specialized knowledge, thereby posing as a major obstacle to its introduction. The construction of a system that allows the introduction of machine learning even with limited information and the reduction of costs for creating high-quality training data are therefore the foremost challenges in the universal use of intelligent systems in practice.
研究の内容
Details of Project
限られた教師データから高精度な予測モデルを自動的に構築する機械学習の基盤技術を研究 Research on Underlying Technology of Machine Learning for Automatically Building High Precision Prediction Models from Limited Training Data
本研究ではこの課題を解決するために、限られた教師データから高精度な予測モデルを自動構築する基盤技術の確立を目指しています。これにより、これまで良質な教師データを大量に集められないという問題から機械学習を導入できなかった領域や、教師データの構築に必要な人的コストや専門知識の不足から導入を断念せざるを得なかった領域まで、機械学習の導入障壁となっていた教師データの整備に関わる様々な課題を根本的に解決できます。
本研究では、三つの観点からこの革新的な基盤技術の確立に取り組んでいます。研究チームはすでに各項目それぞれに世界をリードする成果と評価を得ており、この優位性を維持し加速することで、より突出したコア技術の創出を目指しています。
To solve the challenge, this research aims to establish underlying technology for automatically building high precision prediction models from limited training data. The technology will fundamentally solve diverse problems related to the developing of training data, which has posed as a barrier to the introduction of machine learning, from areas where machine learning could not be introduced due to the failure to gather large amounts of high-quality training data to ones where introduction of machine learning had to be given up due to human costs and lack of specialized knowledge required for the development of training data.
In this research, we plan to establish innovative underlying technology from three perspectives. Our research team has already obtained world-leading achievements and evaluation results for different processes. By maintaining and accelerating this advantage, we aim to create more predominant core technologies.
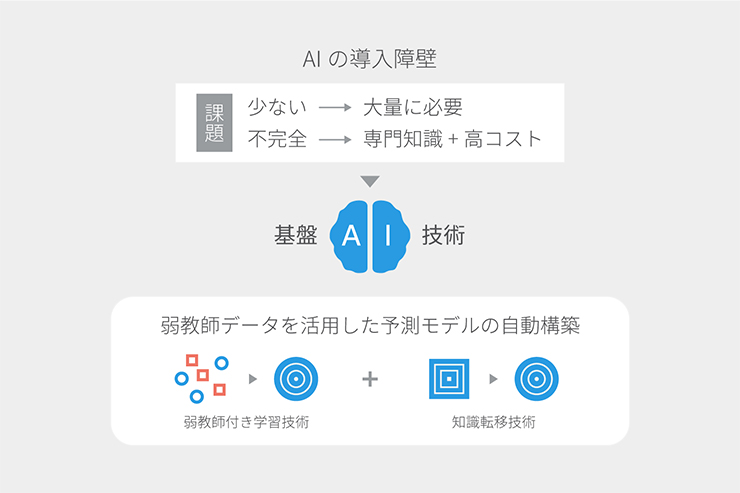
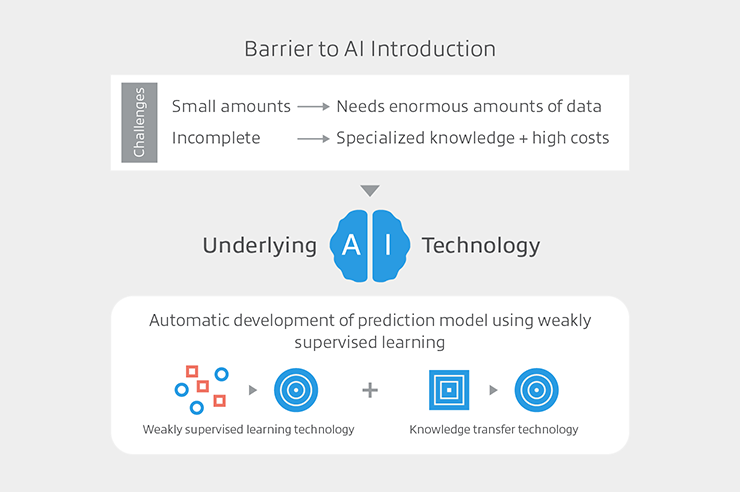
[1]弱教師データを活用した予測モデルの学習理論とアルゴリズムの開発 [1] Development of learning theories and algorithms of prediction models using weakly supervised learning
研究チームでは、これまでにも「弱教師付き学習」と呼ばれる分野において、世界をリードする学習理論の構築と汎用的なアルゴリズムの開発をしてきました。「弱教師付き学習」とは、教師データのラベル情報が不正確であったり、一部のみに付与されていたりする場合の学習手法です。近年では医療情報のようにラベルの収集に多大なコストがかかる場合への強力な解決方法として世界的に注目を集めています。本研究ではこれまでの研究成果をさらに発展させ理論の精緻化を進めることで、最終的には弱教師付き学習の統一的な理論の構築を目指しています。また、この統一理論を実世界で応用可能にするために、汎用的なアルゴリズムの開発や、ノイズや異常値に対応可能な頑健性の追求などの研究開発を推進しています。
Our research team has built world-leading learning theories and general-purpose algorithms in a field called “weakly supervised learning.” Weakly supervised learning is a learning method in which the label information of the training data is inaccurate or given only partially. In recent years, it has attracted interests across the world as a formidable solution for overcoming the massive costs required for collecting labels for medical information, etc. This project thus aims to establish a unified theory on weakly supervised learning by further developing the research results achieved thus far and refining the theory. To enable practical application of the unified theory in the real world, we also promote research and development to build general-purpose algorithms and pursue robustness for dealing with noise and abnormal values.
[2]知識転移の理論とアルゴリズムの開発 [2] Development of knowledge transfer theory and algorithms
もう一つのアプローチとしてドメイン適合という知識転移技術の開発に注力します。ドメイン適合とは、ある領域で学習された予測モデルを、性質の異なるターゲットに転用させる技術です。これにより、シミュレーションにより大量に生成した人工的な知識を、実環境で利用可能な知識として転用することが可能になります。なお、現状のドメイン適合では、ソースとターゲットのカテゴリが一致していなければならないとう強い制約が課題となっていますが、本研究ではこの制約を緩和する技術の研究を推進しています。また、ソースの領域の数や多様性を増やし、その中から適切な知識を選択適合させる技術、さらには[1]弱教師付学習とドメイン適合の組み合わせの手法などを研究開発しています。
Another approach of our focus is the development of knowledge transfer technology called domain adaptation. Domain adaptation is the technique of diverting a prediction model learned in a certain area to a target with different properties. This makes it possible to convert the artificial knowledge generated in large quantities by simulation into knowledge that can be used in real environments. A challenge faced with current domain adaptation is the strong restriction requiring the source and target categories to match. In this project, we promote research on technologies that can ease the restriction. Other research and development endeavors include technologies for increasing the number and diversity of source areas and selecting and adapting appropriate knowledge from them, as well as methods for combining [1] weakly supervised learning and domain adaptation.
[3]高精度な予測モデルの自動構築と応用 [3] Automatic development and application of high precision prediction models
[1]弱教師付き学習や[2]知識転移技術などを組み合わせた統合的な自動学習基盤技術の開発にも取り組みます。機械学習では高精度なモデルを構築するためにモデルの構造決定、データの前処理、パラメータの設定等を適切に行う必要があり、これらの設定を自動的に行う効率的な並列分散基盤の構築を目指しています。特に、[1]弱教師付き学習では、教師データに付与された情報の質(ラベル付き,ラベルなし,ラベルの信頼度,類似度など)による最適なアルゴリズムの選択の自動化、[2]知識転移技術では転移すべき適切な知識の選択の自動化に注力し、さらに、これらの統合手法の開発も行います。
We are also engaged on the development of integrated automatic learning underlying technology combining [1] weakly supervised learning and [2] knowledge transfer technology. To build high precision models in machine learning, it is imperative to appropriately decide model structures, preprocess data, set parameters, etc. We therefore aim to build an efficient parallel distribution processing platform that automatically performs these settings. Particularly, for [1] weakly supervised learning, we will focus on automating the selection of optimum algorithms based on the quality of information assigned to training data (e.g., labeled, unlabeled, label reliability, similarity). For [2] knowledge transfer technology, we will focus on automating the selection of appropriate knowledge to be transferred as well as develop integrated methods for these.
価値・期待
Values / Hopes
本研究プロジェクトが切り開く未来の可能性 Future Possibilities Created by This Project
教師データの整備という機械学習の導入障壁に取り組む本研究は、AI利活用の基盤技術であり、きわめて広い適用範囲があります。本研究によって機械学習導入の新たな可能性を切り開くことで、これまでよりもより広範囲な業種やサービスに機械学習を適用できるようになることを期待しています。また、この基盤技術によって、AIがもたらす知的システムが世の中で汎用的に利用されることで、Beyond AIが目指すよりよい社会の実現に大きく貢献できると信じています。
更に、この基盤技術の確立は科学技術の発展という側面においても大きな成果が期待できます。この技術には、実験回数の制約などから大量の教師データを得ることが困難だった従来の科学研究の方法論を変える可能性があり、様々な自然科学分野において今まで思いもつかなかった新たな知見の獲得につながる可能性を秘めています。
To overcome the training data development barrier to the introduction of machine learning, this project focuses on the development of underlying technology for leveraging artificial intelligence (AI), which can be applied extensively. We hope that our endeavors will pave new avenues for introducing machine learning, and enable machine learning to be applied to an even wider range of industries and services than ever before. We also believe that the underlying technology will significantly contribute to the building of a better society that “Beyond” AI strives for by enabling the wide use of the AI-based intellectual systems around the world.
Furthermore, the underlying technology is expected to produce critical results in terms of the development of science and technology. It has the potential to change conventional methodologies of scientific research, with which it has been difficult to obtain massive amounts of training data due to restrictions on the number of experiments that can be conducted, as well as the potential to help acquire never-imagined knowledge in various areas of natural science.
成果
Research outcome
このプロジェクトの目標は,限られた教師情報から予測モデルを学習する手法と,そのようなモデルを自動構築する手法を開発することである.この目標を達成するために,限られた教師付き情報から学習するための理論とアルゴリズム,知識転移のための理論とアルゴリズム,高精度な予測モデルを自動構築するためのフレームワークを開発する.また,実環境に基づく世界モデルの構築を目指し,時空間モデリングにも取り組んでいる.杉山グループは主に限られた教師情報から学習するための理論とアルゴリズムを担当し,原田グループは主にそれ以外のテーマを担当している.以下に成果の概要を示す.
一つ目のサブ課題では,限られた教師情報から学習するための理論とアルゴリズムの開発を目指している.具体的には,弱い教師情報,雑音を含むラベル,バイアスを持ったデータからの学習に関する研究を行っている.
弱教師付き学習に対しては,利用可能な弱い教師情報だけから分類リスクを不偏推定し,過適合軽減のためのリスク推定量補正を行う統一的な枠組みを開発してきた.これまでに,部分ラベル,一対比較ラベル,一対類似信頼度ラベル,複数セットのラベル無しデータなどから学習を行う手法の開発に成功している.そしてこれらの成果を英語専門書にまとめ, MIT Pressから出版した.さらに,弱教師付き学習法を音声信号強調問題に適用し,その実用的有用性を実証した.
また,ラベル雑音問題に対しては,雑音遷移行列に基づいて分類リスクを不偏的に推定するアプローチを取っている.この枠組みでの主要な技術的課題は,雑音遷移行列の推定である.我々はこの問題の理論的解析を行い,理論的に正当化できるアルゴリズムを開発した.さらに,入力依存雑音というより困難な問題にも取り組み,実用的なアルゴリズムを開発した.加えて,敵対的な雑音への対処法も様々な角度から検討し,ロバストな学習アルゴリズムを開発した.
バイアスを持ったデータに関しては,データを生成する分布が時間と共に変化する連続分布シフト下での学習手法の開発に取り組んでいる.この問題に対して,ラベルシフトと共変量シフトに対して,分布シフトを知ることなく,ラベル無しデータのみから適応的にモデルを更新するオンライン学習手法を開発した.提案手法は,分布シフトがわかっている場合と同レベルの動的リグレットを達成できることを理論的に証明した.
二つ目のサブプロジェクトでは,知識移転のための理論とアルゴリズムの開発を目指している.シミュレーション上で学習したモデルを実環境に適用する場合のように,あるドメインで学習されたモデルを,異なるドメインに適用すると,ドメインの違い(ドメインシフト)により適用先のドメインにおいて期待する予測精度がでない可能性がある.教師なしドメイン適応(Unsupervised Domain Adaptation, UDA)は,モデルが学習されたソースドメインと,ソースとは異なるモデルの適用先であるターゲットドメインの両方の情報を活用することで,ドメインシフト問題を解決する効果的なアプローチである.近年のドメイン適応手法の多くが,ソースドメインでの誤差と周辺分布間の距離を同時に最小化するBen-Davidらの理論をもとにしているが,結合誤差はその推定が困難であるため無視されるのが一般的である.この問題の解決のために,我々は結合誤差の上界に関連する新しい目的関数などを提案した.また,既存のUDA手法のほとんどは,ソースからターゲットドメインに知識を転移する際に,生のソースデータがターゲットドメインの学習時に直接利用可能であることを前提としている.データのプライバシーに関する懸念が高まる昨今において,新規ターゲットドメインでUDA手法を適用する際にソースデータの直接的利用が可能とは限らない.この問題を解決するために,本研究では,ターゲットドメインの学習時にソースデータやソースモデルパラメータを用いない教師なしドメイン適応(SF-UDA)に取り組んでいる.
高精度予測モデルの自動構築フレームワークについては,一般的にモデルの自動構築計算コストが高いことから,モデル自身の効率性が重要となる.そこで,この目標に向けて,低次元で階層構造化されたデータを適切にモデル化できる双曲空間にニューラルネットワークを考案した.このネットワークは,非ユークリッド幾何学である双曲空間を使用し,歪みの少ない木構造を連続的に埋め込むことができる.この研究は,単一の双曲幾何学モデルであるポアンカレ球モデルを用いた統一的な数学的解釈のもとで,ニューラルネットワークの基本的な構成要素を一般化している.また,高速化と消費電力削減のために,スパイク型ニューラルネットワーク(Spiking Neural Network, SNN)にも取り組んだ.SNNはバイナリでイベント駆動型であり,ニューロモルフィックデバイス上で高速かつ超低消費電力で動作させることができる.本プロジェクトでは,画像生成のためにSNNに基づく変分オートエンコーダ(VAE)などを構築した.
画像データはそのまま扱うと数百万次元となり,この次元数に見合う規模の訓練画像を収集することは困難を極める.しかしながら,実世界には3次元の構造,時間を含めると4次元の低次元構造が存在し,この低次元構造を前提知識としてうまく活用することによって,大幅にアノテーションコストの削減につながる可能性がある.そこで,この実世界の低次元構造の活用により必要なデータ数の削減を目標として,部分点群のマッチングとレジストレーション,3次元形状の新しい暗黙的・明示的形状プリミティブ表現手法,スパースな観測画像から多関節物体を対象とした制御可能な輝度場を獲得する研究などに取り組んだ.さらに,輝度場を変形する新しい手法として,自由形状の輝度場変形を提案した.
※詳細については、以下のURL(PDF・英文のみ)をご参照ください。
https://beyondai.jp/contents/wp-content/uploads/2024/01/Research-report_Tatsuya-Harada_202309.pdf
The goal of this project is to develop methods for learning prediction models from limited supervised information and for automatic construction of such models. To achieve this goal, we have developed theories and algorithms for learning from limited supervised information, theories and algorithms for knowledge transfer, and a framework for automatic construction of highly accurate prediction models. In addition, we have worked on spatio-temporal modeling with the goal of constructing world models based on the real environment. Sugiyama group is mainly responsible for developing the theory and algorithms for learning from limited supervised information, while Harada group is mainly responsible for the other topics. An overview of the results is given below.
In the first subproject, we aim to develop theory and algorithm for learning from limited supervision. More specifically, we have been exploring the problems of learning from weak supervision, noisy labels, and biased data.
For weakly supervised learning, we have been developing a unified framework that estimates the classification risk in an unbiased manner only from available weak supervision and corrects the risk estimator to mitigate overfitting. We successfully developed such methods for weakly supervised learning problems from partial labels, pairwise comparison labels, pairwise similarity confidence labels, and multiple sets of unlabeled data. We summarized such a unified framework in a monograph and published it from the MIT Press. Furthermore, we applied a weakly supervised learning method to an audio signal enhancement problem and demonstrated its practical usefulness.
For label-noise problems, we have also been estimating the classification risk in an unbiased manner based on the noise transition matrix. The main technical challenge was estimation of the noise transition matrix since it is non-identifiable without additional assumptions. We conducted theoretical analysis of this problem and developed theoretically justifiable algorithms. Furthermore, we considered an even more challenging scenario of instance-dependent noise and developed practically useful algorithms. In addition, we also considered the problem of coping with adversarial noise. We explored various adversarial scenarios and developed robust learning algorithms.
For data bias, we have been tackling a learning problem under continuous distribution shifts, where data generating distributions are changing over time. For this problem, we developed online learning methods for label shift and covariate shift that adaptively update our model only from unlabeled data without knowing the distribution shift. We theoretically proved that the proposed methods achieve the same dynamic regret as the case where the distribution shift is known.
In the second subproject, we aim to develop theories and algorithms for knowledge transfer. When a model learned in one domain is applied to another domain, e.g. when a model learned in a simulation is applied to a real-world environment, the expected prediction accuracy in the target domain may not be achieved due to domain differences (domain shift). Unsupervised domain adaptation (UDA) is an effective approach to solve the domain shift problem by using information from both the source domain where the model was trained and the target domain where the model is applied. Most recent domain adaptation methods are based on the theory of Ben-David et al. which simultaneously minimizes the error in the source domain and the distance between the marginal distributions, but typically ignores the joint error due to its difficulty in estimation. To solve this problem, we propose a new objective function related to the upper bound of the joint error. In addition, most existing UDA methods assume that the raw source data is directly available during the training of the target domain when transferring knowledge from the source to the target domain. In these days of increasing privacy concerns, direct availability of source data is not always possible when applying UDA methods in a new target domain. To solve this problem, we address Source Data Free Unsupervised Domain Adaptation (SF-UDA), which does not use source data and model parameters during training of the target domain.
Regarding the framework for automatic construction of highly accurate prediction models, we focused on efficient models due to the high computational cost of automatic model construction. To achieve this goal, we introduced hyperbolic space neural networks, which can model hierarchically structured data in low dimensions. These networks use a non-Euclidean geometry, hyperbolic space, which allows continuous embedding of tree structures with low distortion. The study generalizes basic components of neural networks under a unified mathematical interpretation using a single hyperbolic geometric model, the Poincaré ball model. We also worked on spiking neural networks (SNNs) to increase speed and reduce power consumption. SNNs are binary, event-driven, and can run on neuromorphic devices with high speed and low power. In this study, a variational autoencoder (VAE) based on SNNs is constructed for image generation.
Image data as it is would have several million dimensions, and it would be extremely difficult to collect training images on a scale appropriate for that number of dimensions. However, the real world has a 3-dimensional structure, or a 4-dimensional low-dimensional structure if time is included, which can be used as prior knowledge to significantly reduce the cost of annotation. Therefore, with the goal of reducing the amount of data required by exploiting this low-dimensional structure of the real world, we have worked on the matching and registration of partial point clouds, new implicit and explicit shape primitive representation methods for 3D shapes, and the acquisition of controllable radiance fields for articulated objects from sparse observational images and videos. We also proposed a new method to deform the radiance field, namely free-form deformation of the radiance field.
※For more detail, please refer to the URL below.
https://beyondai.jp/contents/wp-content/uploads/2024/01/Research-report_Tatsuya-Harada_202309.pdf