Projects 研究プロジェクト
Projects
人流誘導(ヒトの行動シミュレートと行動に影響を与えるインセンティブ設計の融合) Human Flow Navigation (Combination of Human Behavior Simulation and Incentive Design Affecting Human Behaviors)
構成メンバー
People
- 研究リーダー Project Leader
-
東京大学 工学系研究科 Graduate School of Engineering, The University of Tokyo
- 研究担当者 Researcher
-
-
大知 正直 特任研究員 Masanao Oochi Project Researcher
-
加藤 大造 Taizo Kato
-
國枝 良 Ryo Kunieda
-
坂田 一郎 教授 Ichiro Sakata Professor
-
田中 季晃 Toshiaki Tanaka
-
對馬 清元 Kiyomoto Tsushima
-
News
News
ビデオ
Movie
課題
Challenges
次世代AI都市シミュレーターによる人流再現による都市の課題解決手法の確立 Establishing a new methodology to solve issues in cities by reproducing the flow of people using a “Next-Generation AI City Simulator”
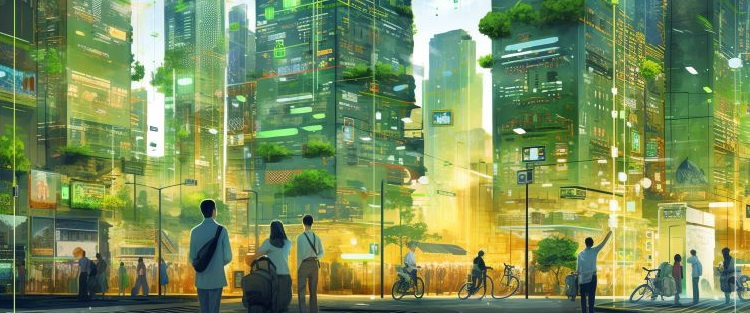
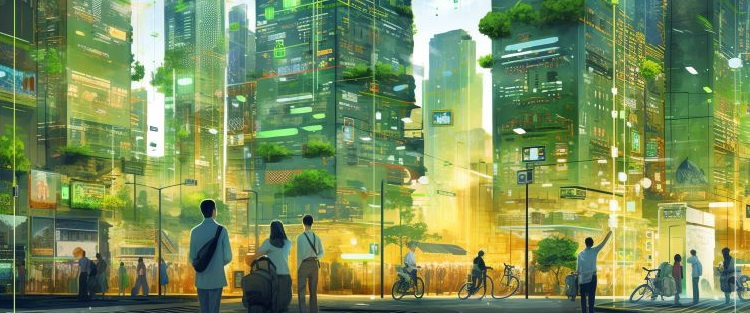
近年、インターネットの普及とデジタル化の発展により、人々の消費行動は大きく変化をしています。人々は自宅から出ることなく世界中の商品やサービスを利用することが可能となり、生活は飛躍的に便利になりました。このデジタル化の流れは、近年のコロナウイルスの影響によって一層加速しています。一方で、リアルな場での消費活動や人の流れに影響を与え、街の賑わいが失われるという新たな課題を生み出しています。
このような背景の下、都市の在り方に大きな変革が求められ、都市のハブであり、商業活動の拠点ともなっている駅前周辺の街づくりや、都市経済を支える商業の賑わいを生むことの重要性は増しています。都市の運営は人々の行動や購買行動へ大きく影響を与え、人々の行動や購買行動が都市の活性化へと直結します。
これまでも街の賑わいを創出することに関して、アカデミックかビジネスかを問わず様々な取り組みや研究が行われてきましたが、本研究においては、特に「人の動き(人流)」に着目し、人流・交通・購買・エネルギー消費など多岐にわたる実データの解析を通じて具体的な都市運営や人の行動をデジタルツインを用いて可視化します。また、データ解析結果を基に、人の行動・都市運営をシミュレートし、人を誘導することで最適な都市運営における解を導出します。
具体的には、人の行動や購買活動が予測・誘導できる「シミュレーター」・「アプリケーション」 の構築を行い、電鉄会社や不動産開発会社などのビジネスの現場において、街や商業施設の課題を可視化し、人流を創出するための最適な施策を実行するための示唆を提供することで、都市の可能性を最大限に引き出すことを目指します。
In recent years, widespread Internet use and digitalization have greatly changed people’s behavior as consumers. People can now access products and services from all over the world without leaving their homes, making their lives significantly more convenient. Moreover, the coronavirus outbreak further accelerated the digitalization of the society. On the other hand, this momentum of digitalization has created a new challenge through commercial areas in cities losing their liveliness as a result of its effect on the consumption activities and the movement of people in physical spaces.
In this context, major changes are required in the way cities are built. Cities are increasingly reliant on commercial activities to support the local economy, and it is becoming more important to develop the areas around train stations as a city hub and commercial center. Effective city management can have a positive impact on the flow of people and their behavior as consumers, which can directly lead to the revitalization of the city.
Following various research and initiatives undertaken by both academia and business communities to create a lively and a bustling city, our research group focuses on the flow of people. We use Digital Twin technology to analyze actual data such as the flow of people, transportation, sales, and energy, and visualize the city management in detail as well as through human behavior. Based on the results of the data analysis, we will develop a solution for optimizing city management by simulating the behavioral paths of people and other factors that affect city management and managing the flow of people. Specifically, our research group aims to build a simulator and an application that can predict and navigate human behavior as well as their purchasing activities. These can be used to identify the challenges facing commercial areas and commercial facilities for businesses such as railway companies and real estate developers. In the end, we will strive to maximize the potential of cities by recommending strategies for implementing optimal measures to attract people to the city.
研究の内容
Details of Project
[1] 現状把握:センシングデータに基づく都市人流可視化 [1] Understanding the current situation: Visualization of human flow in a city based on the sensing data
海老名駅周辺の商業施設エリアを中心にビーコンを設置し、実証実験を実施しました。これにより、都市における人流データを解析し、人流の行動類似性に基づいたクラスターの把握・可視化することが可能となりました。都市における人流の行動特徴の把握や、クラスター別の施策効果検証、イベント施策の効果予測などを通じ、本研究を人流誘導システムの社会実装に向けた基盤として活用することを目指しました。
また、ビーコンデータに加え、改札データ、GPSデータ等の人流センシングデータを組み合わせることで、地域間移動の行動特性データをマクロレベルで把握・可視化しました。
Conducted demonstration tests by installing beacons mainly on the commercial facilities around Odakyu Ebina Station. This demonstration test has made it possible to understand the behavioral characteristics of human flow and visualize clusters based on similarities in behaviors by analyzing actual human flow data in the city. Our goal is to use this research as a foundation for the social implementation of the human flow navigation system, through understanding the characteristics of human flow behavior in cities, verifying the effects of measures by cluster, and predicting the effects of event measures, etc.
Also, captured and visualized macro-level data on behavioral characteristics of inter-regional migration by combining beacons data with human flow sensing data such as ticket gate data and GPS data.
[2] 再現モデル:行動類似性に基づくクラスター再現と成長可能性の評価 [2] Reproduced model: Recreated clusters based on behavioral similarities and growth potential assessment
人流データ分析から人の行動類似性に基づいて訪問客クラスターを分類し、それぞれのクラスターに対して行動再現モデルを構築しました。
「1. 現状把握」の実証実験を通じて取得した人流データから、時間帯や交通モード(鉄道/自動車/自転車/歩行)、居住者/在外者といった環境・状態を考慮することで、「街の回遊行動」や「立寄り行動」等のより現実に近い行動を再現するモデルの開発を目指しました。
また、「街の価値向上に資する人流創出」への寄与度に従って特性指標(回遊指標や訪問頻度等)を設定し、訪問客クラスターごとに成長可能性評価を行いました。
Classified visitors into clusters based on their behavioral similarities by analyzing human flow data and built a behavior reproduction model targeting each cluster.
Tried to develop a model that can reproduce more realistic behaviors such as “migratory behavior in commercial areas” or “strolling behavior”, etc. by considering the situation and status, such as time of day and traffic mode (rail, car, bicycle, walking), and resident/non-resident on the actual data of human flow acquired through the demonstration test for “1. Understanding the current situation”.
Conducted a growth potential assessment on each visitor cluster by setting characteristic indicators (e.g., migration indicators, frequency of visits, etc.) depending on the level of contribution to the “Increase in human flow that contributes to add value to a city”.
Conducted a growth potential assessment on each visitor cluster by setting characteristic indicators (e.g., migration indicators, frequency of visits, etc.) depending on the level of contribution to the “Increase in human flow that contributes to add value to a city”.
[3] 行動再現シミュレーション:行動意思決定モデルとスケジューリングモデルの適用 [3] Behavior reproduction simulation: Adapting behavior and decision-making model and scheduling model
クラスターごとにパラメータを持つ「環境・状態に応じた行動意思決定モデル」と「行動スケジューリングモデル」を与えたエージェントをシナリオに応じて発生させることで、行動再現シミュレーションを行いました。
Conducted a behavior reproduction simulation by generating agents given the “behavior and decision-making model according to the situation and status” and “behavior scheduling model” with parameters for each cluster as per the scenario.
[4] マッチングによる人流誘導探索 :誘導・インセンティブ案探索 [4] Search for human flow navigation by matching: Search for navigation and incentive options
人流に影響を与えると期待される施策として、イベントの実施、サイネージやビーコンを活用した情報発信 による人流誘導などの「インセンティブ施策」をシミュレーション上で再現し、その施策実施の効果推定を行いました。推定結果を基に、マッチングによるより効果的な都市運営のための具体的な施策提案への活用が期待されます。
また、長期的な街の変遷を検証するために、将来のある日における人流やクラスター構成などのパラメータを変化させたシナリオを定義し、ある都市の人流再現を行いました。長期的に街の居住者/訪問者構成や、商業施設の変化、インフラの整備等に拠る街の変化によって、どのように人流や街の賑わいが変化するかをシミュレーションすることで、長期的な街の成長戦略策定への活用を目指しました。
Reproduced incentive measures on the simulator, such as holding customer-attracting events and disseminating information by utilizing signages or beacons, which are expected to induce the flow of people and estimated the effectiveness of each incentive measure. The estimated results are expected to be utilized for designing and suggesting effective incentive measures in detail based on matching for optimal city management.
In order to test the long-term transition of the city, we defined scenarios with varying parameters such as human flow and cluster compositions on a given day in the future to reproduce the human flow in a given city. We aim to use this information to formulate a long-term growth strategy for cities by simulating the change in flow of people and the liveliness of the commercial area according to changes in resident-visitor ratio, the transformation of commercial facilities, and the city’s infrastructures, etc.
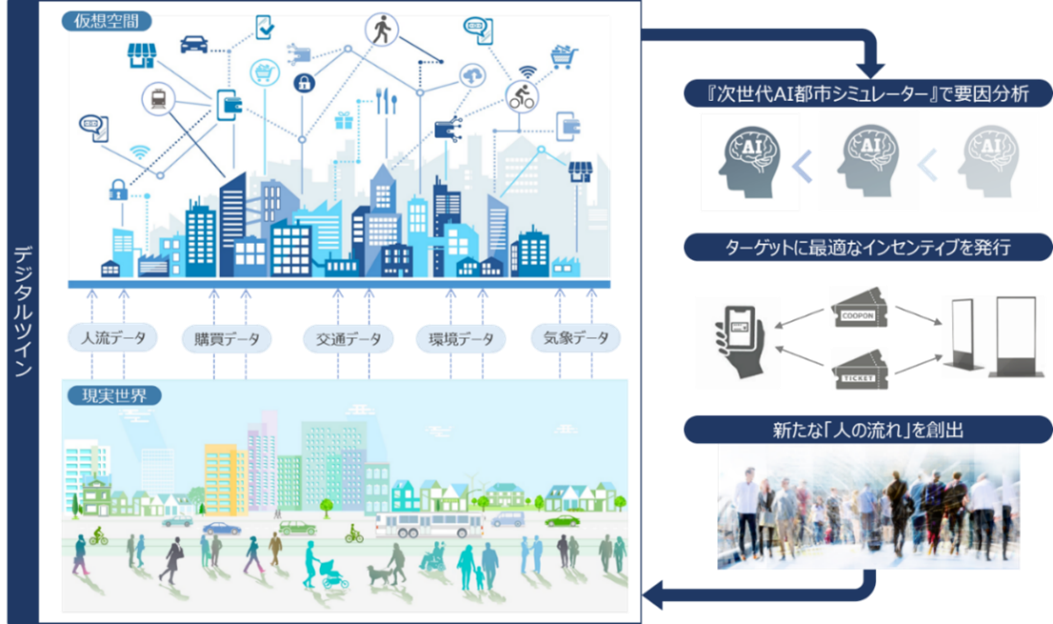
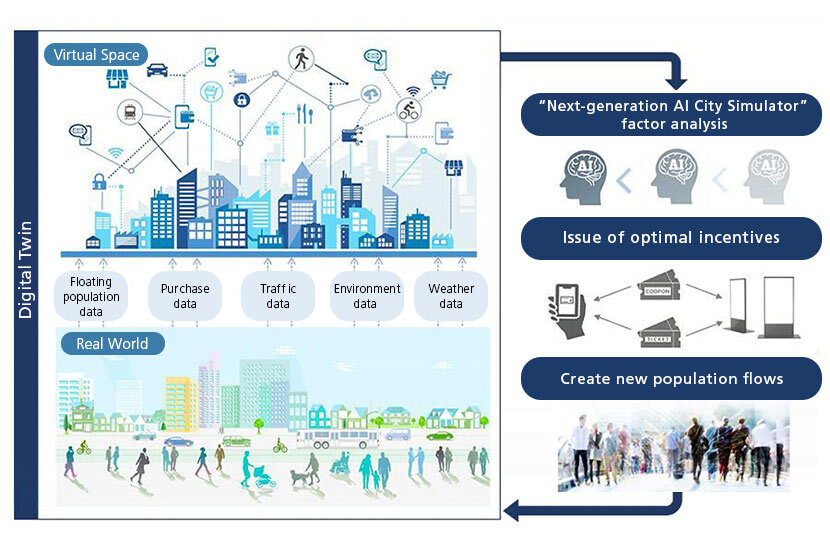
価値・期待
Values / Hopes
本研究が生み出す価値は、都市計画と都市の運営における新たな視点を提供することです。「次世代AI都市シミュレーター」を活用することで、人流を創出するための具体的な施策の効果を予測し、その結果に基づいて最適な都市運営策を提案することを目指します。
人流に影響を与える施策としてのイベント開催やサイネージやビーコンを活用した情報発信などの「インセンティブ施策」の再現と効果推定により、具体的な施策提案が可能となります。人流の創出を通じて、都市全体の活性化や商業活動を促進し、都市の価値向上に貢献することが期待されます。
また、本シミュレーターでは、長期的な都市の変遷をシミュレーションすることも可能となります。具体的には、未来のある日における人流やクラスター構成などのパラメータを変化させたシナリオを設定し、そのシミュレーションの結果から都市の人流がどのように変化するかを予測します。この結果は、長期的な都市の成長戦略の策定や、都市の変化に対応した長期戦略を策定する上で価値のある情報を提供します。
本研究では、人流の可視化やモデル化、そして都市全体における人流のシミュレーションを通じて、これまでの都市運営が「データ駆動型の都市運営」へと移行し、都市全体の活性化と持続可能な発展に寄与できることを期待しています。
The value this research is expected to create is to provide new perspectives in city planning and city management. The Next-Generation AI City Simulator can predict the effectiveness of each incentive measure in detail for inducing flow of people, aiming to suggest an optimal measure for city management based on the simulation results.
By reconstructing incentives that can influence flow of people, such as holding events and disseminating information by signages or beacons, as well as estimating their effectiveness, it becomes possible to suggest concrete incentives. Through the induction of human flow, it is expected to contribute to the revitalization of the city, promote commercial activities, and enhance the value of the city.
The simulator can simulate the long-term change of the city. Specifically, a scenario is set up in which parameters such as human flow and cluster composition are varied on a given day in the future. The results of the simulation are then used to predict how the human flow in the city will change. This information is valuable for cities to formulate long-term growth strategies and as city plans that can adapt to the long-term changes in the city.
This research project is expected to change conventional city management into data-driven city management by visualizing and modeling human flow and simulating human flow throughout a city. This will lead to the revitalization and sustainable development of the entire city.