Projects 研究プロジェクト
Projects
複合AIによる問題解決手法 Problem-solving methods using multiple AI models
構成メンバー
People
- 研究リーダー Project Leader
-
東京大学 素粒子物理国際研究センター International Center for Elementary Particle Physics, The University of Tokyo
- 研究担当者 Researcher
-
-
齊藤 真彦 助教 Masahiko Saito Assistant Professor
-
森永 真央 特任助教 Masahiro Morinaga Project Assistant Professor
-
GANGULY Sanmay 特任助教 Sanmay Ganguly Project Assistant Professor
-
Events
Events
- 第10回 Beyond AI 研究推進機構 サイエンスカフェ 動画を公開 The 10th Institute for AI and Beyond Science Café Video is now available.
- 第1回 ML@HEPワークショップを開催しました The 1st ML@HEP Workshop was held on July 8,9, 2022.
- 第30回研究セミナー「最新の画像認識モデルの素粒子物理学実験への応用」 The 30th Research Seminar: Application of recent image recognition models to particle physics experiments
- 第20回研究セミナー「The deep relation between LHC physics and machine learning」 20th Research Seminar: The deep relation between LHC physics and machine learning
ビデオ
Movie
課題
Challenges
AIを便利な「ツール」からより「知能」らしく Making AI more “intelligent” beyond a useful “tool”
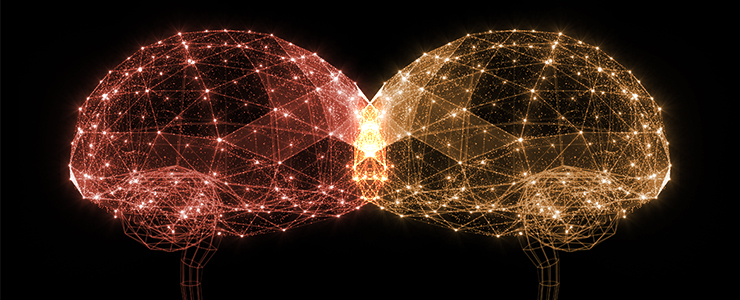
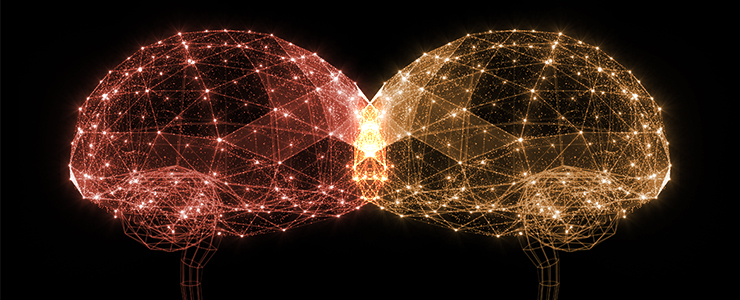
素粒子実験分野でもAIを活用した研究が進んでいますが、あくまで1つ1つの問題に対して適用するに過ぎず、「知能」というよりも、単なる便利な「ツール」という位置づけにとどまっています。私たちの究極の研究テーマは、この状況を一歩でも前進させ、より「知能」らしいふるまいができるAIとはどういったものか、を追求することです。素粒子実験の問題からスタートし、私たちを含めた多くの研究者が一丸となって行っている多段階型の問題処理を如何に機械学習の枠組みに落とし込み、従来以上の成果が得られるか、ということに挑戦します。この研究を通して「ツール」を超えたより「知能」らしいAIの可能性を模索します。
Although research using AI has been advancing in the field of particle physics experiments, AI is only applied to one problem at a time and is regarded as a useful “tool” rather than a form of “intelligence.” Our ultimate research theme is to take this situation one step further and pursue how AI can behave more like intelligence. Starting with the problems of particle physics experiments, we will take on a challenge to incorporate multi-stage problems, for which many experimental particle physicists including ourselves have been working together, into a framework of machine learning with expecting better results than before. Through this research, we will explore the potential of AI that looks like “intelligence” rather than “tool.”
研究の内容
Details of Project
問題解決に向けて調整・統括の役割をAIに取り入れる Integrating the roles of adjustment and supervision into AI to solve problems
人間が直面する問題は、一見シンプルに見えているにもかかわらず、実は複数の問題が重なり合っているため、個別の問題を解決した先に最終的な問題が解決できる、というような複合・多段階型の問題であることが多くあります。私たちの社会では、こういった問題に対して、一つの大きな問題の全容を把握し、解決に向けて種々の問題の間を調整・統括するような役割をもつ人を置くことで、問題解決に至るまでの効率を高め、質の高い解決策を得ることが出来るようになります。このような仕組みをAIの中に取り入れることで、AIの効率化を図るとともに、従来のAIができなかったような成果を得ることが期待出来ます。
Although problems we encounter seem to be simple at first glance, they are often complex and multi-staged. Since an actual problem involves subproblems, the final solution may be reached only after all the subproblems have been solved. To tackle such a problem in our society, we improve efficiency to solve issues and derive high-quality solutions by assigning people who can understand the whole picture of the problem, and adjust and control its subproblems. Incorporating such a mechanism into AI is expected to improve the efficiency of AI and achieve results that conventional AI could not.
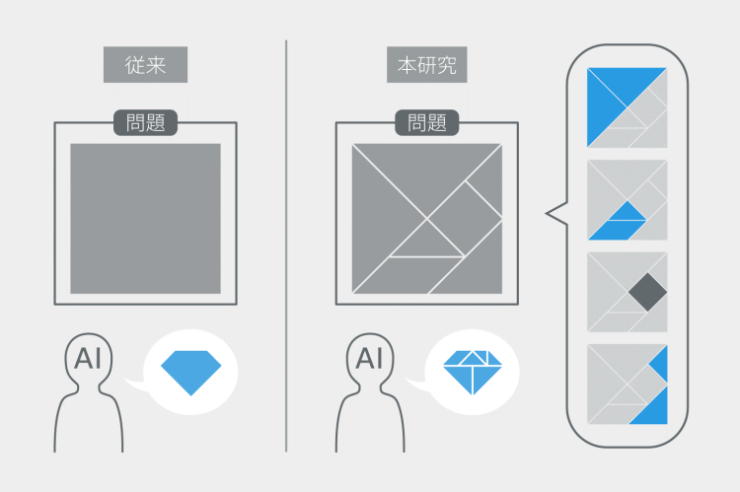
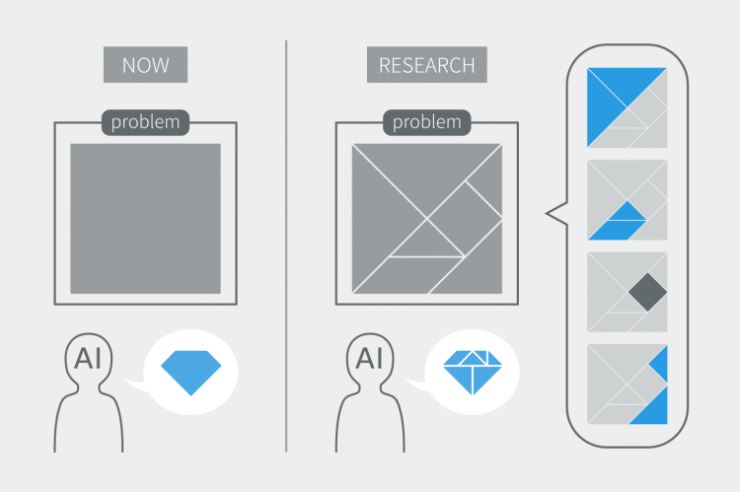
【1】AIをAIが学習する「Multi-AI」の開発 [1] Developing “Multi-AI” where AI learns from AIs
個々の問題を調整し全体を指揮するAIフレームワークとして、多段階で、機能毎に構成されたAI群(=機械学習モデルの集合)を統括AIが学習し、問題に合わせて適宜選択するような「Multi-AI」の研究開発を行っています。これまで、多段の機械学習モデルを接続し、最適化するフレームワークの開発を進めてきました。今後、多段化による複雑な損失関数を取り扱うための高度なパラメータ最適化などの研究を行います。さらに、多段化された機械学習モデル間のデータから人間が理解できるような情報を獲得する可能性、学習済みの機械学習モデルの再活用(多段化された転移学習等)による問題解決などの研究を進めていきます。
As an AI framework for coordinating individual subproblems with controlling the whole process, we have been working on the research and development of “Multi-AI,” in which the control AI learns from a group of AIs (i.e., a set of machine learning models) configured for each function and then selects an appropriate AI from them for each subproblem. So far, we have developed a framework for connecting, optimizing and selecting multi-step machine learning models. In the future, we will conduct advanced research on parameter optimization to handle complex loss functions in the multi-step processing. We will also investigate the feasibility of getting information that can be understood by humans from intermediate data between machine learning models, and solving problems by reusing trained machine learning models (e.g., multi-staged transfer learning).
【2】Multi-AIの応用可能性を実証する [2] Demonstrating the feasibility of applying Multi-AI
並行して、このようなMulti-AIの仕組みを、素粒子物理国際研究センターで行っている素粒子物理実験のデータに適応します。私たちの実験では、数100ペタバイト(1ペタバイトは、1000兆バイト)というビッグデータを扱っています。そのようなビッグデータから新しい素粒子などの発見、たとえば、2012年のヒッグス粒子発見を行うためには多段型の問題を解く必要があります。Multi-AIが有効に機能すれば、素粒子物理分野の研究が進むのはもちろん、Multi-AIの応用可能性が実証されることになります。さらに、素粒子物理以外の一般社会における課題等にも応用し、本研究で開発したフレームワークの有効性を示していくことを目指します。
Concurrently, we will apply this Multi-AI framework to the data of particle physics experiments conducted at the International Center for Elementary Particle Physics, the University of Tokyo. In our experiments, we handle big data of several hundred petabytes (1 petabyte is 1000 trillion bytes). To discover new subatomic particles, etc., from such big data, we need to solve multi-stage problems, as shown in the discovery of the Higgs boson in 2012. If working effectively, Multi-AI will not only advance research in the field of particle physics but also demonstrate its applicability. Furthermore, we aim to demonstrate the effectiveness of the framework developed in this project by applying it to problems in general society other than particle physics.
価値・期待
Values / Hopes
AIを理解する Understand AI
大きな問題を1つの機械学習モデルとして解くAIと細分化して小さな問題として解くAIには人間の解釈性という点では後者に分があると考えています。細分化・多段化することによる(少々の)性能ロスがあったとしても、解釈性が担保できれば、素粒子実験のような自然科学のみならず実社会においても説明が求められる課題に対してAIの活用が進むと考えています。
Considering two types of AI: one that solves a large problem as a single end-to-end machine learning model and the other that solves subproblems by subdividing a problem, we think that the latter is better in terms of human interpretability. Despite the (slight) performance loss that comes with subdividing and multi-staging, if interpretability can be ensured, such an AI could be used in order to solve problems that require explanation for their solutions in both natural science, such as particle physics experiments, and the real world.
成果
Research outcome
複合AIの第一歩として、複数の機械学習モデルの接続・選択ができるフレームワークの開発を行いました。ベースとなる技術は、機械学習モデルのネットワークアーキテクチャを最適化するNeural Architecture Search(NAS)で、本研究で開発したMultiMLフレームワークでは、3つの接続・選択手法:DARTS, SPOS-NAS, ASNG-NASを実装しました。
素粒子実験シミュレーションデータを用いて、2つのタスクを連結してタウ粒子対に崩壊するヒッグス粒子とZボソン粒子を識別する問題をベンチマークに、このフレームワークの開発に取り組みました。前段タスクはタウ粒子の運動量を求める学習、後段タスクはタウ粒子対がヒッグス粒子由来かZボソン粒子由来かを識別する学習となり、後段タスクは前段タスクの結果を受け取って識別を行います。まず、それぞれのタスクに対して複数の機械学習モデルを準備します。MultiMLフレームワークは個々のモデルで学習を行いながら、複数の組み合わせから最適な1組を選択します。単にハイパーパラメータチューニングとしても利用できますが、全く違う機械学習モデルも候補にできます。
課題としては、タスクの接続時のデータの受渡しによる情報ロスがあることです。前段タスクの出力、我々のベンチマークの場合、運動量を後段タスクに直接渡すのではなく、潜在パラメータとして受け渡すことで解決しました。前段タスクの問題は潜在パラメータを入力とした別の浅いネットワークを追加することで学習します。これで、後段タスクへの情報ロスを最小限にし、全体性能を回復させました。このフレームワークを利用することで、個別の問題に特化して開発された機械学習モデルを、より大きな問題の中の一部として容易に組み込み最適化することが可能になります。
並行して、素粒子実験のデータ解析に関する最新の機械学習技術の応用研究を実施しました。転移学習による少ない学習データでの事象識別、ファインマン図とグラフネットワークを組み合わせた事象識別、Normalizing flowを導入した異常検知を用いた新粒子探索、Vision Transformerを用いたヒッグス粒子や大半径ジェット識別、グラフネットワークを用いたトップ粒子識別、Diffusion modelを用いたハドロン化モデリングなどの研究を行いました。これらは個別の問題に特化した機械学習であり、将来、MultiMLフレームワークを用いた素粒子実験データ解析の部品として利用することが可能になります。
As the first step of our “Multi-AI”, we developed a framework that can connect and select multiple machine learning models. The base technology is Neural Architecture Search (NAS), which optimizes the network architecture of machine learning models, and our framework called “MultiML” developed in this research has three connection and selection methods: DARTS, SPOS-NAS, and ASNG-NAS.
Using experimental particle physics simulation data, we developed this framework by benchmarking the problem of identifying Higgs and Z bosons that decay into tau pairs. In this problem, two tasks are defined and connected. The former task is learning to calculate the momentum of the tau particle, and the latter task is learning to identify whether the tau particle pair is produced from the Higgs particle or the Z boson particle. The latter task uses the result of the former task as input and performs discrimination. First, we prepare some machine-learning models for each task. Then, the MultiML framework selects the optimal set from multiple combinations while learning individual models. This framework can be used simply for hyperparameter tuning, but it can also handle completely different machine-learning models as candidates.
As a problem, there is information loss due to data transfer at the task connection. To solve this problem, the output of the previous task, in our benchmark case, the momentum is not passed directly to the subsequent task, but latent-space parameters are passed. The previous task is learned by adding another shallow network with latent parameters as input. This minimized information loss to subsequent tasks and restored overall performance. By using this framework, machine learning models developed for specific problems can be easily incorporated and optimized as part of a larger problem.
We also conducted applied research on the latest machine learning technology for data analysis of elementary particle experiments: event identification with a small amount of training data by transfer learning, event identification by combining Feynman diagrams and graph networks, new particle search in anomaly detection using normalizing flow, Higgs and large radius jet identification using Vision Transformer, top-quark identification using graph network, and modeling of jet hadronization using a diffusion model. These are machine learning for specific problems, and in the future, it will be possible to use them as components of our data analysis using the MultiML framework.
※詳細については、以下のURL(PDF)をご参照ください。
https://beyondai.jp/contents/wp-content/uploads/2023/05/Research-result_Junichi-Tanaka_202303.pdf
※For more detail, please refer to the URL below.
https://beyondai.jp/contents/wp-content/uploads/2023/05/Research-result_Junichi-Tanaka_202303.pdf