Projects 研究プロジェクト
Projects
脳情報再現による次世代AI開発プロジェクト Development of next generation AI by modeling brain information
構成メンバー
People
- 研究リーダー Project Leader
-
東京大学 大学院医学系研究科 / 国際高等研究所 ニューロインテリジェンス国際研究機構 Graduate School of Medicine / International Research Center for Neurointelligence (IRCN), The University of Tokyo
- 研究担当者 Researcher
-
-
合原 一幸 副機構長・特別教授 Kazuyuki Aihara University Professor / Deputy Director
-
中山 英樹 教授 Hideki Nakayama Professor
-
根東 覚 特任准教授 Satoru Kondo Project Associate Professor
-
高橋 真有 講師 Mayu Takahashi Junior Associate Professor
-
吉田 盛史 講師 Takashi Yoshida Lecturer
-
村上 知成 助教 Tomonari Murakami Assistant Professor
-
西尾 奈々 特任助教 Nana Nishio Project Assistant Professor
-
関谷 敬 特任助教 Hiroshi Sekiya Project Assistant Professor
-
橋本 昂之 助教 Takayuki Hashimoto Assistant Professor
-
木村 梨絵 特任助教 Rie Kimura Project Assistant Professor
-
竹内 大吾 特任講師 Daigo Takeichi Project Lecturer
-
徐 牧原 特任研究員 Xu Muyuan Project Researcher
-
Li Yang 特任研究員 Li Yang Project Researcher
-
Minh Duc Vo 特任研究員 Minh Duc Vo Project Researcher
-
岸野 文昭 特任研究員 Fumiaki Kishino Project Researcher
-
北島 奈美 特任研究員 Nami Kitajima Project Researcher
-
菊田 浩平 特任研究員 Kohei Kikuta Project Researcher
-
- 研究協力者 Research Collaborator
- Cao Lang Cao Lang 福田 裕太 Yuta Fukuda 陳 夢円 Mengyuan Chen 田 文軒 Wenxuan Tian 安田 裕之 Hiroyuki Yasuda 香取 勇一 Yuichi Katori 徳田 慶太 Keita Tokuda 梶田 真司 Masashi Kajita 馬場 雅基 Masaki Baba 呉 宇軒 Yuxuan Wu Kuanchao Chu Kuanchao Chu 鹿島 大河 Taiga Kashima 張 芷瑜 Zhang Zhiyu 堀本 秋水 Syusui Horimoto 敷町 怜愛 Reia Shikimachi 畑 悠貴 Yuki Hata 肖 中洋 XIAO ZHONGYANG 田村 浩人 Hiroto Tamura 勝又 海 Kai Katsumata Jiaxuan Li Jiaxuan Li 山下 優樹 Yuki Yamashita Yi-Pei Chen Yi-Pei Chen 味岡 雄大 Takehiro Ajioka 須藤 育太郎 Ikutaro Suto 根本 野乃子 Nonoko Nemoto Junwen Mo Junwen Mo
Events
Events
- 第71回研究セミナー 「Short-Term Memory-Enhanced Ising Machine Based on Spin Cluster Glass」 The 71st Research Seminar: "Short-Term Memory-Enhanced Ising Machine Based on Spin Cluster Glass "
- 第65回研究セミナー 「The Impact of Retrieval-Augmented Generation in Image Captioning」 The 65th Research Seminar: "The Impact of Retrieval-Augmented Generation in Image Captioning "
- 第49回研究セミナー 「リカレントニューラルネットワークを用いた神経ダイナミクス解析」 The 49th Research Seminar: Analysis of Neural Dynamics Using Recurrent Neural Networks
- 第42回研究セミナー「マウス視覚系の神経ネットワーク形成において視床-皮質回路が果たす役割」 The 42nd Research Seminar: Thalamo-cortical template circuits for the formation of hierarchical and parallel pathways in the mouse visual system
News
News
- 【プレスリリース】脳自身が生み出す活動と外界からの入力による活動を大脳神経回路が分離する新しいメカニズムを解明 [Press Release] Elucidating the Mechanism by which Neural Circuits in the Cerebral Cortex Separate Spontaneous Brain Activity from Sensory-Evoked Activity
- 【プレスリリース】脳のゆらぎを取り入れてAIを安全にする ――深層ニューラルネットワークの隠れ層にゆらぎを導入し脆弱性を軽減―― [Press Release] Making AI secure by introducing random noise that mimics brain neurons ――Reduces its vulnerability against some adversarial examples by injecting random noise in the hidden layers of deep neural networks――
- 【プレスリリース】大脳神経回路形成の新戦略――大脳皮質の多数の領野を結ぶ結合を効率よく作るための並列モジュール戦略を解明 Press Release: New strategy for cerebral neural network formation――Parallel modules strategy which enables the brain to efficiently form numerous interareal connections of the many cortical areas during development.
ビデオ
Movie
課題
Challenges
人工知能の誤認識のリスク Risks of Misrecognitions by AI
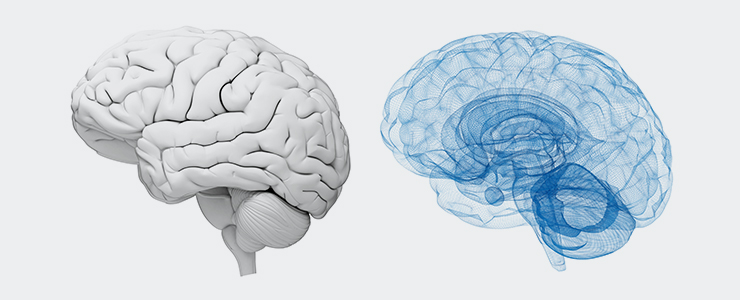
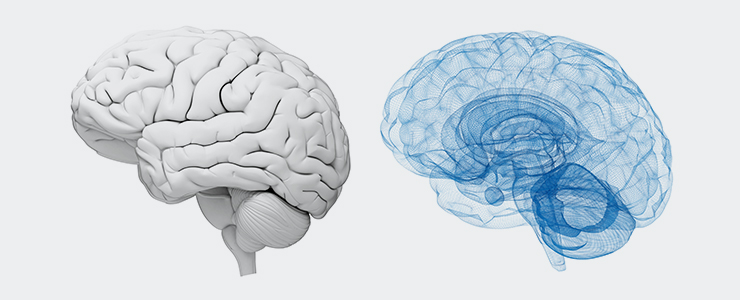
現在の人工知能を用いた画像認識では、原理的に誤認識が起こる可能性が避けられません。
例えば、人間には明らかにパンダと見える絵に、わずかなノイズを加えるだけで、全く別の物体と誤認識することが知られています。近年、深層学習を用いた人工知能の発展は著しく、人間に近い性能で物体の認識を行うことができるようになってきており、今後、さまざまな分野で人工知能の活用が期待されます。しかし、例えば自動運転や医療画像の診断のような分野では、このような誤認識は人の命を左右する事態になりかねず、致命的な欠点になります。
The risks of misrecognition are, in principle, unavoidable in existing image recognition technologies using artificial intelligence (AI). For example, AI is known to falsely recognize a picture that apparently looks like a panda to humans for something completely different when a small amount of noise is added. The recent years have witnessed the remarkable development of AI using deep learning. AI has been increasingly able to recognize objects at performance levels close to those of humans and is expected to be applied to a range of areas. However, such misrecognition errors pose as a fatal drawback that can affect human lives in fields such as autonomous driving and medical image analysis.
研究の内容
Details of Project
頑強かつ柔軟な人工知能の開発 Development of Robust and Flexible AI
本研究では、脳で実際に行われている情報処理を人工知能上に再現することにより、誤認識をしない頑強かつ柔軟な人工知能の開発を目指しています。
上述のような人工知能の誤認識の可能性をなくすためには、私たち人間を含む動物がどのように目で見た視覚情報を処理しているのかを解明することが役立つと考えています。すなわち、脳で実際に行われている情報処理を解明して、これを人工知能上に再現することができれば、現在の人工知能の原理では得られない、誤認識をしない頑強かつ柔軟な人工知能の開発が期待されます。
このため、本研究では、大脳皮質の多数の領域にある細胞の神経活動を網羅的に計測し、計測した脳活動を人工知能に再現することにより、現在の人工知能の原理では得られない頑強かつ柔軟な人工知能の構築を目指しています。
In this research, we aim to develop robust and flexible AI preventing misrecognition errors by reproducing in AI the information processing carried out by our brain. The elucidation of how animals, including humans, process visual information may prove useful for eliminating any risks of misrecognition by AI as described above. This means that if we can elucidate the information processing actually performed in the brain and reproduce it in AI, we may be able to develop robust and flexible AI preventing misrecognition, which is unrealizable by the current principle of AI.
To this end, this research aims to comprehensively measure the neural activity of cells in many regions of the cerebral cortex and reproduce the measured brain activity in AI to build such innovative AI.
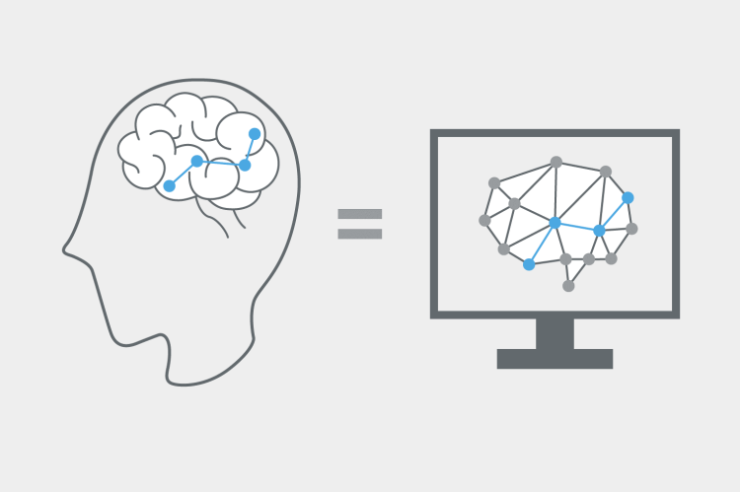
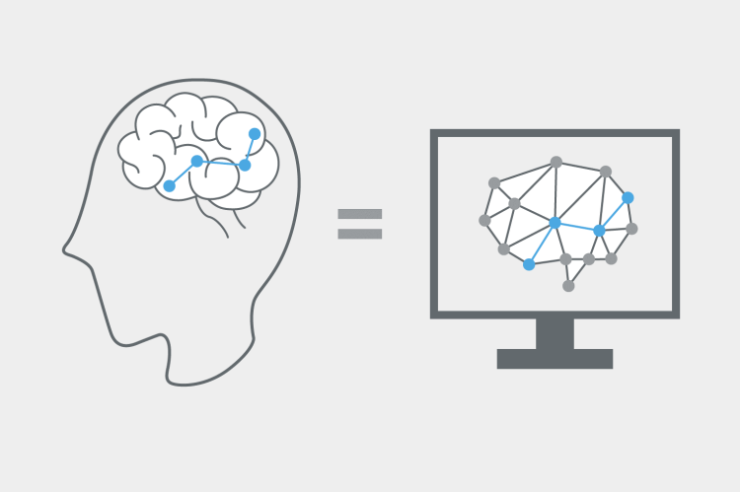
【1】大脳皮質の神経活動の網羅的な計測 [1] Comprehensive measurement of neural activity in the cerebral cortex
研究チームでは、大脳皮質の多数の領域にある細胞の神経活動を網羅的に計測する技術を開発してきました。
私たち人間を含む動物の脳における視覚情報の処理は、大脳皮質の多数の領域で、階層的に行われていることが知られています。このため、脳で実際に行われている情報処理を理解するためには、脳の特定の領域における情報処理に加えて、領域間の情報処理を把握することが重要です。
研究チームは、「二光子カルシウムイメージング」という、生きた動物の脳内の神経細胞の活動を、二光子顕微鏡という特殊な顕微鏡を用いて観察する技術を独自に開発してきました。この方法を用いると、数ミリ四方の範囲から数万個以上の 1 個 1 個の細胞の活動を観察することができます。
本研究ではこれらの技術をさらに発展させ、大脳皮質の多数の領域にある細胞の神経活動を網羅的に計測することを目指します。
Our research team has developed a technology to comprehensively measure neuronal activity in cells in multiple areas of the cerebral cortex. It is known that visual information processing in the brains of animals including humans is performed hierarchically in many areas of the cerebral cortex. To understand the actual mechanisms of the processing in the brain, it is important to investigate such mechanisms in specific regions and between different regions of the brain.
Our team has developed a unique “two-photon calcium imaging” technique for observing the activity of neuron cells in the brain of living animals using a special two-photon microscope. Using this method, we can observe the activity of tens of thousands of cells in an area of several millimeters square, even the activity in each cell.
In this research, we aim to further develop these technologies and measure the neural activity of cells in many areas of the cerebral cortex more comprehensively.
【2】神経細胞の活動を人工知能上に再現 [2] Reproduction of neuron cell activity in AI
研究チームではまた、さまざまな画像に対する視覚野の神経細胞の活動を深層ニューラルネットワークに写し取ることで、神経細胞の活動をコンピュータ上で詳細に解析する手法を開発してきました。
具体的には以下のようなステップで、神経細胞の活動を解析することができます。(1)動物に見せた画像と同じ画像を深層ニューラルネットワークに入力し、その画像に対する神経細胞の活動を教師信号として、深層ニューラルネットワークに画像に対する神経細胞の活動を学習させます。(2)このニューラルネットワークの出力をなるべく大きくするような画像をコンピュータ上で生成することによって、このニューラルネットに写し取った神経細胞の反応特性を推定します。具体的には、まずランダムなノイズ画像を用意し、その画像に対するニューラルネットワークの出力をより大きくするように画像の輝度値を更新します。その更新された画像に対して、ニューラルネットワークの出力をより大きくするように、再度画像の更新を何度も繰り返すことにより、ニューラルネットワークの出力を最大にする画像をコンピュータ上で生成しました。出来上がった画像は、このニューラルネットに写し取った神経細胞の活動を最大にするような画像であり、神経細胞の反応特性を示していると推測されます。
研究チームは、この手法をマウス一次視覚野の神経細胞の解析に適用することで、さまざまな一次視覚野の性質を説明できることを示しました。上記の方法で大脳皮質の多数の領域にある細胞の神経活動を網羅的に計測したデータにこの手法を用いることにより、脳の階層間での情報の抽出、分析、統合を網羅的に解析することを目指します。さらに、これらの視覚情報処理を担う神経細胞の活動を人工知能上に再現し、従来の人工知能では使われていなかった神経活動のゆらぎや、トップダウン結合・再帰性結合を含めて、人工知能上に写し取ることにより、現在の人工知能の原理では得られない頑強かつ柔軟で動的な脳型の人工知能を構築することを目指します。
Our research team has also developed a technique for analyzing the activity of neuron cells in detail on computer by copying the activity of neuron cells in the visual cortex from various images onto deep neural networks.
Specific steps for analyzing the activity of neuron cells include: (1) Input the image shown to an animal into the deep neural network, and have the network learn the activity of neuron cells, taking the activity responding to the image as teaching signals; and (2) on a computer, generate an image where the output of the neural network has been maximized to estimate the response characteristics of the neuron cell responses copied to the neural network. Specifically, prepare a random noise image and update the brightness value of the image so that the output of the neural network for that image becomes larger. Repeat the updating process several times to maximize the output of the neural network. The finally updated image maximizes the activity of neuron cells copied to the neural network and is presumed to indicate the response characteristics of the neuron cells.
Our team has demonstrated that the technique can be applied to the analysis of neuron cells in the mouse primary visual cortex to explain the properties of various primary visual cortex. Applying the data obtained by measuring the neural activity of cells in many regions of the cerebral cortex using the technique, we further aim to comprehensively analyze the extraction, analysis, and integration of information between layers of the brain. Furthermore, by reproducing the activity of the neurons responsible for these visual information processing on artificial intelligence, and by copying the fluctuation of neural activity, including top-down and recursive connections, which have not been used in conventional artificial intelligence, onto artificial intelligence, we aim to construct a robust, flexible, and dynamic brain-type artificial intelligence that cannot be obtained with current artificial intelligence principles.
価値・期待
Values / Hopes
本研究プロジェクトが切り開く未来の可能性 Future Possibilities Created by This Project
本研究が目指す人間の脳機能を実装した人工知能は、現在の人工知能とは原理的に全く異なるものであり、極めて広範な産業分野に影響を及ぼすと期待しています。
従来は原理的に不可避だった誤認識が生じないため、例えば、医療現場でのX線、CT、MRIなどの画像診断や、病理組織診断に応用することにより、万が一の場合の診断ミスを防ぐことができます。また、自動運転に適用しても誤認識に起因する致命的な事故につながるおそれがありません。
さらに、この研究成果を活用するにより、人の表情から感情を認識することが将来的に可能になれば、家事や介護などの対人サービスの分野でもこれまで以上に人に寄り添ったサービスを提供できるようになると期待しています。
上記の例に限らず、本研究は、現実社会のより複雑な状況に応用可能な人工知能の新たな可能性を切り開くものだと信じています。
The AI equipped with human brain functions is the target of our research. It is completely different from present AI in principle and is expected to contribute to a very wide range of industries.
Since misrecognition, which has been unavoidable from the principle of existing AI, can be avoided, our innovative AI can be applied to, for instance, diagnostic imaging such as X-rays, CT, and MRI, and to histopathological diagnosis in the medical scene, thereby preventing possible diagnostic errors. Furthermore, its application to autonomous driving will be unlikely to cause fatal accidents resulting from misrecognition.
It is hoped that the results of our research will also one day enable AI to recognize emotions from human facial expressions. If this is the case, more person-focused services will be provided in the face-to-face area such as housework and nursing care.
In addition to the aforementioned examples, we believe that our research will open up new possibilities for AI that can be applied to more complex situations in the real world.
成果
Research outcome
■ 大脳皮質にある多数の領野をつなぐ結合が、発達時に精密に形成されるメカニズムを解明しました(Murakami et al., 2022, Nature)。ヒトの大脳皮質には180にも及ぶ多数の領野が存在し、それらの間には数千通りにおよぶ精密な結合が存在し、階層的かつ並列的な回路が形成されています。本研究では、これらの大脳皮質の多数の領野間をつなぐ結合が形成されるメカニズムを解明しました。これらの大脳皮質の領野をつなぐ結合が形成される前に、網膜と一次視覚野および多数の高次視覚野をつなぐ経路が先に形成され、この経路を伝播する網膜由来の自発活動により、網膜の場所をあらわす情報が一次視覚野および高次視覚野に伝えられ、これが教師信号となって、一次視覚野および多数の高次視覚野の対応する場所を結ぶ精密な結合が形成されることが示されました。これらの領野間を結ぶ精密な神経回路による階層的かつ並列的な情報処理は、私たちの脳が複雑かつ汎用的な知性を獲得するための基盤となっており、この原理を人工知能に応用し、汎用的な人工知能の開発に役立つ可能性が考えられます。
■ 人間など動物における情報処理の性質をAIに取り入れることで、敵対的攻撃に対する脆弱性を克服する新たな方法を開発しました(Ukita & Ohki, 2023, Neural Networks)。動物の神経細胞において知られている性質の中でも特に、神経細胞が持つゆらぎに着目しました。動物の視覚情報処理においては、情報処理の入力部に位置する網膜だけでなく、その後段に位置する脳においてもゆらぎが存在します。ゆらぎは、外界の情報が持つばらつきにも対応できる回路を学習する上で重要と考えられます。そこでまず、隠れ層に敵対的攻撃を行う新しい手法を考案しました。その後、隠れ層にゆらぎを導入することで、今回の敵対的攻撃に対する脆弱性を軽減することに成功しました。この方法を用いることで、より人間などの動物の振る舞いに近いAIが作成できる可能性が高まると考えられます。さらに、学習に基づく量子化を画像空間や隠れ層(特徴空間)で行うことにより、推論時の未知ノイズに対して頑健な画像認識手法の開発を行いました(Hataya et al., IJCNN 2022; Kishida et al., ICPR 2022)。
■ 霊長類の一次視覚野に、色と形の情報がどのように表現されているのかを明らかにしました(Chatterjee et al., Nature Commun., 2021)。一次視覚野のblob(直径約300ミクロンの色情報に特化したモジュール)で赤・緑と青・黄の情報が合流しますが、そのとき完全に混ざりあうのではなく部分的に混ざりあうことにより中間色を含む色相の情報についてのマップが形成されることを明らかにしました。さらにblobでは低空間周波数(物体の表面など)の色情報が表現され形の情報は表現されていませんが、blobのすぐ外側では、色情報と形情報が組み合わさって表現されていること、そのさらに外側では高空間周波数の形情報だけが表現され色情報は表現されていないことを明らかにしました。現在の深層学習では、R, G, Bの情報をそのまま入力しているが、空間周波数によっては、形情報、色情報が不要なチャンネルもあり、それを考慮することによって霊長類に近い物体認識を行う人工知能の開発に寄与する可能性があります。
■ マウスの脳から計測された自発活動のデータを用いて、再帰型ニューラルネットワークを学習させ、脳の自発活動のダイナミクスをニューラルネット上に再現することを目的として、Full-FORCE法を改良した新たな学習法Hidden-FORCE法を開発しました。そして、本手法を応用して、マウスの脳から計測された自発活動のデータを用いて、データ駆動に再帰型ネットワークを学習させ、全脳の自発活動データの一部をリカレントニューラルネットワークで再現・予測することに成功しました。
■ 大脳皮質の動的自発活動を数理モデル化する基礎モデルとして、ノイズを含むStuart-Landau発振子からなる非線形ネットワークの平均場解析手法などの開発を行いました(Li et al., 2022)。さらに、サルを使った神経科学実験で、カテゴリー情報を用いた論理的な思考過程に関係する神経活動を発見するとともに、その理論モデルを構築することで論理的思考が脳内で行われるメカニズムについて解析しました(Hosokawa et al., 2022)。この成果は、人間の高次な認知機能のさらなる理解につながるほか、論理的思考を可能とする人工知能の開発への将来の応用が期待されます。
■ 次世代の動的AI構築に向けて、 様々な複雑系大規模データの変化を予測する新しいAI予測理論である「オートリザバー計算」手法を提案し、実際の複雑系から観測される多数の観測ビッグデータの空間情報を特定のターゲット変数の予測に活用するための数理的手法を構築しました。この「オートリザバー計算」は、予測が重要な意味を持つ多様な複雑系の予測精度向上が期待出来ます(P.Chen et al., Nature Communications, 2020)。
■ ニューロシンボリックAIの開発を目指して、離散的な操作を含む先見的な構造や知識をトップダウンに入れるモジュールを組み込んだニューラルモジュールネットワークを開発しました。これは、帰納と演繹の融合という人工知能の一大テーマに挑むものであります。まず、離散的な問題を連続の問題へ緩和するアプローチを用い、微分可能探索により従来の画像認識におけるデータ拡張探索手法を数百倍高速することに成功しました(Hataya et al., ECCV, 2020)。また、離散的な探索をそのまま実行する、より汎用性の高いアプローチについても研究を進めています。このアプローチでは、モジュールの組み合わせを表す中間的なラベルの探索を行う必要がありますが、我々は効率的なグラフ表現を用いた高速な探索手法を開発しました(Wu et al., ACCV, 2020)。さらに、トランスフォーマに外部知識グラフを接続することにより、与えられた画像の未来の状況を予測して記述する画像予測キャプショニングを世界で初めて実現しました(Vo et al., CVPR 2023)。
■ 画像生成についても、敵対的生成ネットワーク(GAN)について多くの成果を得ています。まず、少量データや不完全データからのGANの学習手法の開発を体系的に進めています(Katsumata et al., ICASSP 2021; Katsumata et al., CVPR 2022; Katsumata et al., WACV 2024)。また、識別ネットワークの改良や中間分布の導入による画像生成品質の改善(Yang et al., Access 2022; Yang et al., WACV 2023)、プルーニングによるGANモデルの圧縮 (Vo et al., WACV 2022)なども実現しました。さらに、これらの基礎技術を基盤とし、多言語手書き文字生成(Jan et al., ACMMM 2021; Jan et al., ICDAR 2023)、画像からの自然なテキスト削除(Jan et al., WACV 2020)、ストーリー画像列生成 (Chen et al., EMNLP 2022)などの応用アプリケーションの開発も多数行いました。
References
1. Huu Hoang, Eric J. Lang, Yoshito Hirata, Isao T. Tokuda, Kazuyuki Aihara, Keisuke Toyama, Mitsuo Kawato , Nicolas Schweighofer. Electrical coupling controls dimensionality and chaotic firing of inferior olive neurons. PLoS Computational Biology 16(7): e1008075 (2020. 7. 30)
2. Pei Chen, Rui Liu, Kazuyuki Aihara, and Luonan Chen: "Autoreservoir computing for multistep ahead prediction based on the spatiotemporal information transformation," Nature Communications, Vol.11, Article No.4568, pp.1-15 (2020) (2020.9.11)
3. Soumya Chatterjee, Kenichi Ohki, R Clay Reid. “Chromatic micromaps in primary visual cortex” Nature Communications., .Vol.12: 2315. doi: 10.1038/s41467-021-22488-3 (2021.04.19).
4. Rui Liu, Jiayuan Zhong, Renhao Hong, Ely Chen, Kazuyuki Aihara, Pei Chen, and Luonan Chen, "Predicting Local COVID-19 Outbreaks and Infectious Disease Epidemics based on Landscape Network Entropy," Science Bulletin, pp.2265-2270 (2021). (2021.3.26:online, 2021.11.30:print)
5. Murakami T, Matsui T, Uemura M, Ohki K. Modular strategy for the development of hierarchical visual network in mice. Nature 608:578-585. (2022).
6. Yang Li, Jifan Shi, and Kazuyuki Aihara: "Mean-Field Analysis of Stuart-Landau Oscillator Networks with Symmetric Coupling and Dynamical Noise," Chaos, Vol.32, No.6, Article No.063114, pp.1-23 (2022). (2022.6.3: online)
7. Takayuki Hosokawa, Muyuan Xu, Yuichi Katori, Munekazu Yamada, Kazuyuki Aihara and Ken-Ichiro Tsutsui: "Monkey Prefrontal Single-Unit Activity Reflecting Category-Based Logical Thinking Process and Its Neural Network Model," Journal of Neuroscience, Vol.42, No.33, pp.6380-6391 (2022). (2022.7.8: online)
8. Rui Yang, Raphael Shu, and Hideki Nakayama: "Improving Noised Gradient Penalty with Synchronized Activation Function for Generative Adversarial Networks," IEICE Transactions on Information and Systems, Vol.E105-D, No.9, pp.1537-1545, (2022). (2022.9.1: online)
9. Jumpei Ukita, Kenichi Ohki Adversarial attacks and defenses using feature-space stochasticity Neural Networks 167 875-889 2023
10. Ryuichiro Hataya, Zdenek Jan, Kazuki Yoshizoe, and Hideki Nakayama. Faster AutoAugment: Learning Augmentation Strategies using Backpropagation, In Proceedings of the 16th European Conference on Computer Vision (ECCV), 2020. (2020.8.23)
11. Kai Katsumata, Hideki Nakayama. Semantic Image Synthesis from Inaccurate and Coarse Masks. Proceedings of the 46th IEEE International Conference on Acoustics, Speech, and Signal Processing (ICASSP), pp.2285-2289 (2021). (2021.6.11)
12. Jan Zdenek and Hideki Nakayama. JokerGAN: Memory-Efficient Model for Handwritten Text Generation with Text Line Awareness. Proceedings of the 29th ACM International Conference on Multimedia (ACMMM), pp.5655-5663 (2021). (2021.10.23)
13. Duc Minh Vo, Akihiro Sugimoto, and Hideki Nakayama: "PPCD-GAN: Progressive Pruning and Class-Aware Distillation for Large-Scale Conditional GANs Compression," In Proceedings of the IEEE Winter Conference on Applications of Computer Vision (WACV), pp.2436-2444, 2022. (2022.1.4)
14. Kai Katsumata, Duc Minh Vo, and Hideki Nakayama: "OSSGAN: Open-Set Semi-Supervised Image Generation," In Proceedings of the Conference on Computer Vision and Pattern Recognition (CVPR), pp.11185-11193, 2022. (2022.6.19)
15. Ryuichiro Hataya and Hideki Nakayama: "DJMix: Unsupervised Task-agnostic Image Augmentation for Improving Robustness of Convolutional Neural Networks," In Proceedings of the International Joint Conference on Neural Networks (IJCNN), pp.1-8, 2022. (2022.7.18)
16. Ikki Kishida and Hideki Nakayama: "Pixel to Binary Embedding Towards Robustness for CNNs," In Proceedings of the International Conference on Pattern Recognition (ICPR), pp.2279-2285, 2022. (2022.8.21)
17. Hong Chen, Duc Minh Vo, Hiroya Takamura, Yusuke Miyao, and Hideki Nakayama: "StoryER: Automatic Story Evaluation via Ranking, Rating and Reasoning," In Proceedings of the Conference on Empirical Methods in Natural Language Processing (EMNLP), pp.1739-1753, 2022. (2022.12.7)
18. Jan Zdenek and Hideki Nakayama. Handwritten Text Generation with Character-specific Encoding for Style Imitation. Proceedings of the 17th International Conference on Document Analysis and Recognition (ICDAR). 2023.8.31.
19. Rui Yang, Duc Minh Vo, and Hideki Nakayama. Indirect Adversarial Losses via an Intermediate Distribution for Training GANs. Proceedings of the IEEE/CVF Winter Conference on Applications of Computer Vision (WACV), pp.4641-4650, 2023.1.3.
※詳細については、以下のURL(PDF・英文のみ)をご参照ください。
https://beyondai.jp/contents/wp-content/uploads/2024/03/Research-report_Kenichi-Ohki_202309.pdf
■ We elucidated the mechanism by which the connections connecting the many areas of the cerebral cortex are precisely formed during development (Murakami et al., 2022, Nature). The human cerebral cortex contains as many as 180 areas, which are connected by thousands of precise connections, forming hierarchical and parallel circuits. In this study, we elucidated the mechanisms underlying the formation of connections among these many areas of the cortex. We found that the pathways connecting the retina to primary and many higher-order visual areas are formed first, before the connections connecting the cortical areas are formed. The retina-derived spontaneous activity propagating along these pathways conveys retinal location information to the primary and higher- visual areas, which serve as instruction signals to form precise connections between the corresponding locations in the primary and many higher-visual areas. Hierarchical and parallel information processing by precise neural circuits connecting these areas is the basis for the complex and general intelligence of our brain, and this principle may be applied to artificial intelligence to develop general artificial intelligence.
■ We developed a new way to overcome vulnerability to adversarial attacks by incorporating the properties of information processing in humans and other animals into AI (Ukita & Ohki, 2023, Neural Networks). Among the properties known in animal neurons, we focused on the variability that neurons possess. In visual information processing in animals, variability exists not only in the retina, which is located at the input of information processing, but also in the brain, which is located at the later stage of information processing. Variability is thought to be important for training circuits that can respond to variations in information from the outside world. Therefore, we first devised a new method of adversarial attack on the hidden layer. Then, by introducing variability in the hidden layer, we succeeded in reducing the vulnerability to this adversarial attack. This method is expected to increase the possibility of creating an AI that more closely resembles the behavior of humans and other animals. Furthermore, we developed an image recognition method that is robust against unknown noise during inference by performing learning-based quantization in image space and hidden (feature) space (Hataya et al., IJCNN 2022; Kishida et al., ICPR 2022).
■ We clarified how color and shape information is represented in the primate primary visual cortex (Chatterjee et al., Nature Communication, 2021). We showed that red, green, blue, and yellow information merges in the blob (a color-specific module about 300 microns in diameter) of the primary visual cortex, and that this partial blending, rather than complete blending, forms a map of hue information, including intermediate colors. Furthermore, we clarified that the blob represents color information at low spatial frequencies (such as the surface of objects) and no shape information, while just outside the blob, color information and shape information are represented in combination, and further outside the blob, only shape information at high spatial frequencies is represented and no color information is represented. In current deep learning, R, G, and B information is input as it is, but depending on the spatial frequency, shape and color information may be unnecessary in some channels, and taking this into account may contribute to the development of artificial intelligence for object recognition similar to that of primates.
■ Using the data of spontaneous activity measured from mouse brains, we developed a new learning method, Hidden-FORCE method, which is an improvement of Full-FORCE method, to reproduce the dynamics of spontaneous brain activity on a recurrent neural network. Then, by applying this method, we successfully reproduced and predicted the whole-brain spontaneous activity data with a recurrent neural network by training the recurrent network in a data-driven manner using the spontaneous activity data measured from the mouse brain.
■ As a basic model for mathematical modeling of dynamic spontaneous activity in the cerebral cortex, we developed a mean-field analysis method for nonlinear networks consisting of Stuart-Landau oscillators with noise (Li et al., 2022). Furthermore, we discovered neural activity related to the process of logical thinking using categorical information in neuroscience experiments using monkeys, and analyzed the mechanism of logical thinking in the brain by constructing a theoretical model of this activity (Hosokawa et al., 2022). These results are expected to lead to further understanding of higher cognitive functions in humans and to future applications in the development of artificial intelligence that enables logical thinking.
■ For the construction of a next-generation dynamic AI, we proposed new AI prediction theory for predicting changes in various complex large-scale data, and developed a mathematical method for utilizing spatial information of numerous big data observed from real complex systems for the prediction of specific target variables. This "auto-reserver computation" can be expected to improve the prediction accuracy of diverse complex systems for which prediction is important (P.Chen et al., Nature Communications, 2020).
■ Aiming to develop a neuro-symbolic AI, we developed “Neural Module Network” that incorporates discrete operation modules for incorporating and utilizing some kind of known structure and knowledge in neural networks in a top-down manner. This challenges the major theme of artificial intelligence; the fusion of induction and deduction. First, using an approach that relaxes a discrete problem into a continuous problem, we succeeded in making the conventional data expansion search method in image recognition several hundred times faster by introducing differentiable search technique (Hataya et al., ECCV, 2020). We also studied a more general approach that performs discrete search as is. While this approach requires searching for intermediate labels representing combinations of modules, we developed a fast search method using an efficient graph representation (Wu et al., ACCV, 2020). Furthermore, by connecting an external knowledge graph to the transformer, we realized the world's first anticipation captioning, which predicts and describes the future situation of given images (Vo et al., CVPR 2023).
■ In image generation, we obtained many results on adversarial generative networks (GANs). First, we systematically developed methods for training GANs from small amounts of data and incomplete data (Katsumata et al., ICASSP 2021; Katsumata et al., CVPR 2022; Katsumata et al., WACV 2024). We also improved the quality of image generation by improving discriminative networks and introducing intermediate distributions (Yang et al., Access 2022; Yang et al., WACV 2023), and compressing GAN models through pruning (Vo et al., WACV 2022). Furthermore, based on these basic technologies, we developed a number of applications such as multilingual handwritten text generation (Jan et al., ACMMM 2021; Jan et al., ICDAR 2023), natural text removal from images (Jan et al., WACV 2020), story image sequence generation (Chen et al., EMNLP 2022), and many other applications.
References
1. Huu Hoang, Eric J. Lang, Yoshito Hirata, Isao T. Tokuda, Kazuyuki Aihara, Keisuke Toyama, Mitsuo Kawato , Nicolas Schweighofer. Electrical coupling controls dimensionality and chaotic firing of inferior olive neurons. PLoS Computational Biology 16(7): e1008075 (2020. 7. 30)
2. Pei Chen, Rui Liu, Kazuyuki Aihara, and Luonan Chen: "Autoreservoir computing for multistep ahead prediction based on the spatiotemporal information transformation," Nature Communications, Vol.11, Article No.4568, pp.1-15 (2020) (2020.9.11)
3. Soumya Chatterjee, Kenichi Ohki, R Clay Reid. “Chromatic micromaps in primary visual cortex” Nature Communications., .Vol.12: 2315. doi: 10.1038/s41467-021-22488-3 (2021.04.19).
4. Rui Liu, Jiayuan Zhong, Renhao Hong, Ely Chen, Kazuyuki Aihara, Pei Chen, and Luonan Chen, "Predicting Local COVID-19 Outbreaks and Infectious Disease Epidemics based on Landscape Network Entropy," Science Bulletin, pp.2265-2270 (2021). (2021.3.26:online, 2021.11.30:print)
5. Murakami T, Matsui T, Uemura M, Ohki K. Modular strategy for the development of hierarchical visual network in mice. Nature 608:578-585. (2022).
6. Yang Li, Jifan Shi, and Kazuyuki Aihara: "Mean-Field Analysis of Stuart-Landau Oscillator Networks with Symmetric Coupling and Dynamical Noise," Chaos, Vol.32, No.6, Article No.063114, pp.1-23 (2022). (2022.6.3: online)
7. Takayuki Hosokawa, Muyuan Xu, Yuichi Katori, Munekazu Yamada, Kazuyuki Aihara and Ken-Ichiro Tsutsui: "Monkey Prefrontal Single-Unit Activity Reflecting Category-Based Logical Thinking Process and Its Neural Network Model," Journal of Neuroscience, Vol.42, No.33, pp.6380-6391 (2022). (2022.7.8: online)
8. Rui Yang, Raphael Shu, and Hideki Nakayama: "Improving Noised Gradient Penalty with Synchronized Activation Function for Generative Adversarial Networks," IEICE Transactions on Information and Systems, Vol.E105-D, No.9, pp.1537-1545, (2022). (2022.9.1: online)
9. Jumpei Ukita, Kenichi Ohki Adversarial attacks and defenses using feature-space stochasticity Neural Networks 167 875-889 2023
10. Ryuichiro Hataya, Zdenek Jan, Kazuki Yoshizoe, and Hideki Nakayama. Faster AutoAugment: Learning Augmentation Strategies using Backpropagation, In Proceedings of the 16th European Conference on Computer Vision (ECCV), 2020. (2020.8.23)
11. Kai Katsumata, Hideki Nakayama. Semantic Image Synthesis from Inaccurate and Coarse Masks. Proceedings of the 46th IEEE International Conference on Acoustics, Speech, and Signal Processing (ICASSP), pp.2285-2289 (2021). (2021.6.11)
12. Jan Zdenek and Hideki Nakayama. JokerGAN: Memory-Efficient Model for Handwritten Text Generation with Text Line Awareness. Proceedings of the 29th ACM International Conference on Multimedia (ACMMM), pp.5655-5663 (2021). (2021.10.23)
13. Duc Minh Vo, Akihiro Sugimoto, and Hideki Nakayama: "PPCD-GAN: Progressive Pruning and Class-Aware Distillation for Large-Scale Conditional GANs Compression," In Proceedings of the IEEE Winter Conference on Applications of Computer Vision (WACV), pp.2436-2444, 2022. (2022.1.4)
14. Kai Katsumata, Duc Minh Vo, and Hideki Nakayama: "OSSGAN: Open-Set Semi-Supervised Image Generation," In Proceedings of the Conference on Computer Vision and Pattern Recognition (CVPR), pp.11185-11193, 2022. (2022.6.19)
15. Ryuichiro Hataya and Hideki Nakayama: "DJMix: Unsupervised Task-agnostic Image Augmentation for Improving Robustness of Convolutional Neural Networks," In Proceedings of the International Joint Conference on Neural Networks (IJCNN), pp.1-8, 2022. (2022.7.18)
16. Ikki Kishida and Hideki Nakayama: "Pixel to Binary Embedding Towards Robustness for CNNs," In Proceedings of the International Conference on Pattern Recognition (ICPR), pp.2279-2285, 2022. (2022.8.21)
17. Hong Chen, Duc Minh Vo, Hiroya Takamura, Yusuke Miyao, and Hideki Nakayama: "StoryER: Automatic Story Evaluation via Ranking, Rating and Reasoning," In Proceedings of the Conference on Empirical Methods in Natural Language Processing (EMNLP), pp.1739-1753, 2022. (2022.12.7)
18. Jan Zdenek and Hideki Nakayama. Handwritten Text Generation with Character-specific Encoding for Style Imitation. Proceedings of the 17th International Conference on Document Analysis and Recognition (ICDAR). 2023.8.31.
19. Rui Yang, Duc Minh Vo, and Hideki Nakayama. Indirect Adversarial Losses via an Intermediate Distribution for Training GANs. Proceedings of the IEEE/CVF Winter Conference on Applications of Computer Vision (WACV), pp.4641-4650, 2023.1.3.
※For more detail, please refer to the URL below.
https://beyondai.jp/contents/wp-content/uploads/2024/03/Research-report_Kenichi-Ohki_202309.pdf